What is APCER (Attack Presentation Classification Error Rate)?
Author: teresa_myers | 01 Jul 2024On June 6th, 2024, the FATE Morph research publication by NIST entitled NISTIR 8292 DRAFT SUPPLEMENT was updated. This testing is regularly carried out on facial recognition solutions to analyze their performance in presentation attack detection like face morphing. Two primary quantities; APCER & BPCER are reported that are the determinants of the accuracy and robustness of a facial recognition tool.
In this knowledge base, we will explain the first quantity metric i.e. APCER.
What is APCER?
APCER is an acronym for Attack Presentation Classification Error Rate which is defined as the frequency of a biometric facial recognition tool with which it wrongly accepts a biometric presentation attack as a genuine face presentation. It is also termed the ‘Morph Miss Rate’ in NIST research and testing publications.
How is APCER Calculated?
It is calculated in the following equation:
The number of morph images wrongly classified as bona fide images is divided by the total number of actual morph attack images.
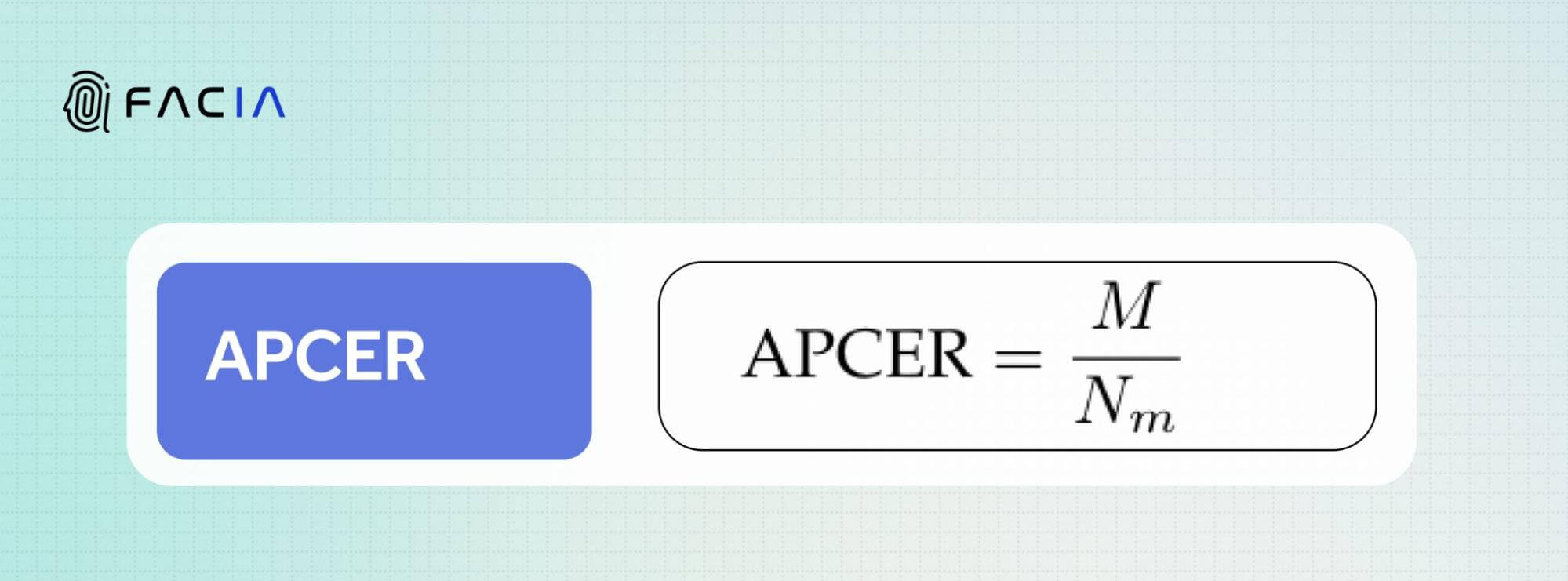
APCER vs. BPCER
As per NIST findings, it is not possible to calculate APCER alone until and unless:
- A Presentation Attack Image curation is completed
- BPCER values are set, fixed & kept below such as:
- APCER @ BPCER = 0.01 in Fate Morph Detection (For Face Morphing and Impersonation attacks)
- APCER @ BPCER = 0.0001 in FRVT PAD API (for all other Presentation attack types)
What is the Ideal APCER?
Based on the above set value, APCER values are calculated for different biometric identity solution providers by NIST.
Look at the statistical explanation of APCER’s calculation above a threshold below:
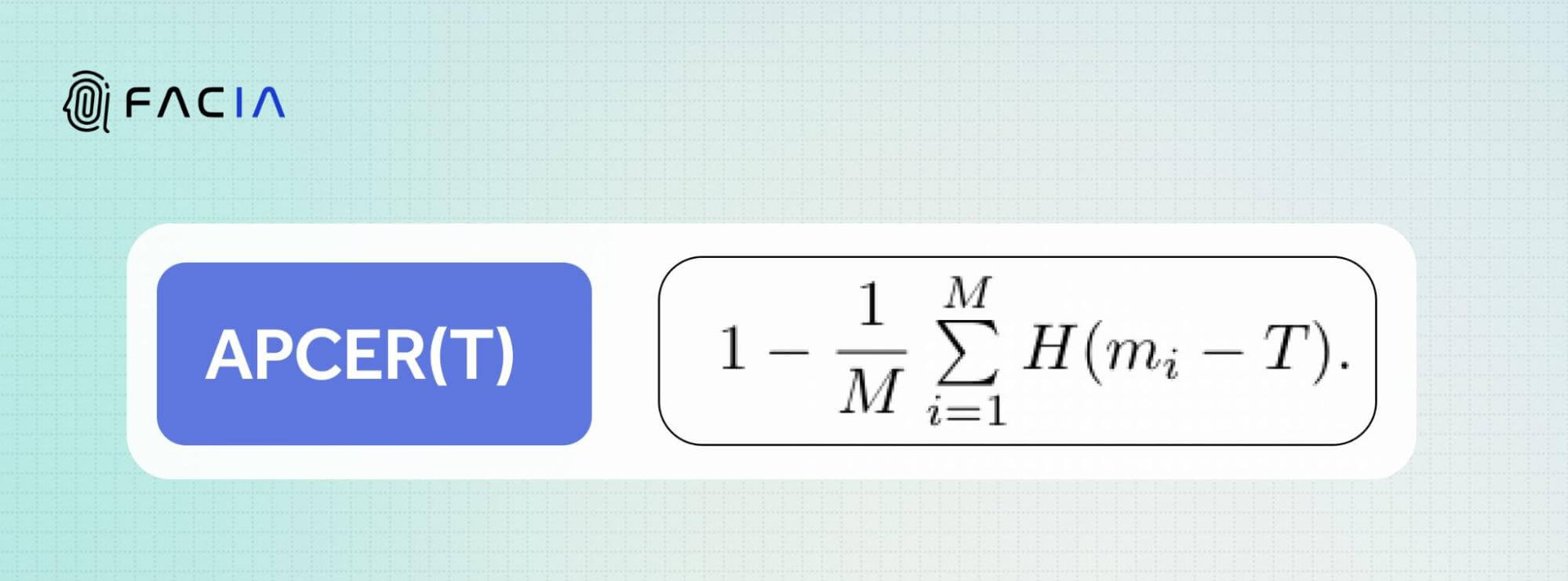
Here is the analytical summary of APCER in biometrics values under different image sets presented to the algorithm tested by NIST:
Single-Image Morphing Detection
A single image (morphed or bona fide) is presented to the algorithm.
Here are the summarized results:
Input Image Type | Tier 1 & 2 | Data Sets | APCER | APCER@BPCER = 0.1 | APCER @BPCER = 0.01 |
Morphed or Bona fide | Low-Quality Morphing | Online Morphing Tool (Website) | Min: 0.016 Max: 0.998 Average: 0.47 | Min: 0.000 Max: 1.000 Average: 0.53 | Min: 0.094 Max: 1.000 Average: 0.75 |
Morphed or Bona fide | Low-Quality Morphing | Global Morph | Min: 0.000 Max: 1.000 Average: 0.33 | Min: 0.000 Max: 1.000 Average: 0.48 | Min: 0.000 Max: 1.000 Average: 0.6035 |
Morphed or Bona fide | Automated Morphing | Local Morph | Min: 0.000 Max: 1.000 Average: 0.354 | Min: 0.000 Max: 1.000 Average: 0.434 | Min: 0.000 Max: 1.000 Average: 0.712 |
Morphed or Bona fide | Automated Morphing | Locally Morphed Colorized Average | Min: 0.000 Max: 1.000 Average: 0.413 | Min: 0.000 Max: 1.000 Average: 0.378 | Min: 0.000 Max: 1.000 Average: 0.683 |
From the above findings, it is clear that few biometric system algorithms have achieved 0.000 APCER under specific conditions. However, the dataset contains 22 algorithmic submissions under each data set and tier. This shows that solutions need to improve their accuracy levels to minimize presentation attack acceptance rate.
A further summary of test results related to APCER vs. BPCER is given in the knowledge piece of BPCER.
Impact of APCER Value in Biometric Facial Recognition
It is evident that if APCER in biometrics is high there is a high chance of identity theft attempts going undetected resulting in bigger threats like:
- Account Takeover fraud
- Financial scams
- Crypto fraud and theft
- Money Laundering
It is important to keep the face presentation attack detection in place by using an AI-powered facial recognition solution that is compliant with NIST standards in achieving the lowest possible error rates. For this purpose, the facial recognition tool must be regularly tested on NIST metrics with improvements in algorithms to reach a perfect zero. In this way, the solution will become spoof-proof for Biometric PAD (Presentation Attack Detection) using facial recognition. Moreover, the solutions need to improve their liveness detection feature to ensure the prevention of presentation attacks & their error rates well before time.