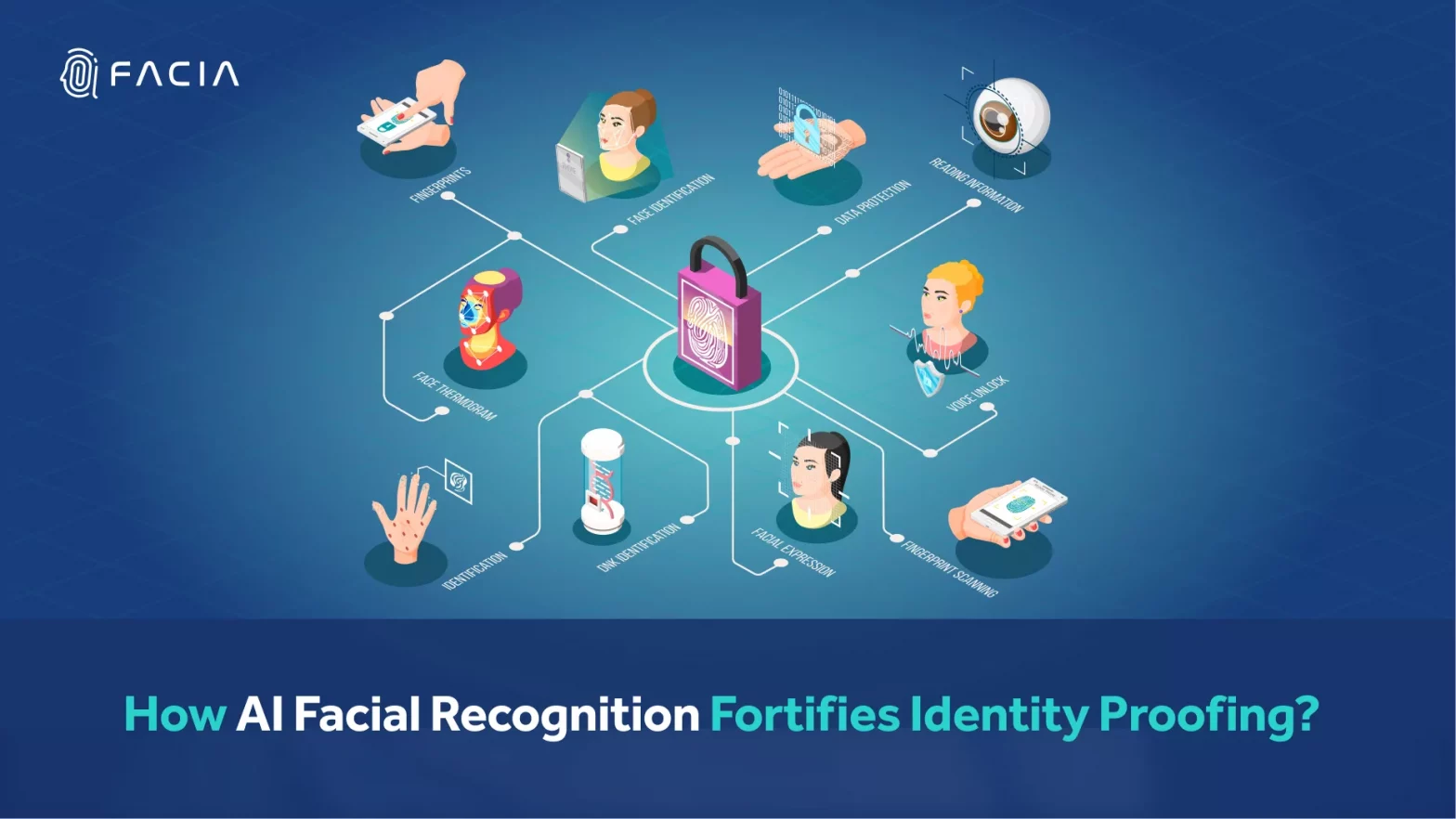
How AI Facial Recognition Fortifies Identity Proofing?
Author: teresa_myers | 25 Apr 2024In This Post
We witness today what we fantasized about decades ago. Yes! AI is here and its pervasive dominance and influence across multiple industries is inevitable. AI is reshaping digital identities strikingly and international bodies like NIST are continuously developing new algorithms in response to its ever-evolving capabilities in detecting identity fraud attempts.
AI Facial Recognition is expected to grow to US $7 billion this year whereas the global facial recognition systems market is said to grow to US $9.9 billion. By 2025, 90% of new cars will have facial recognition systems embedded for security and safety purposes. (Gitnux)
In this blog, we will explore the technical relationship between AI Facial Recognition and Identity Verification and how these two become an unparalleled proactive system for protecting your identities digitally.
After Reading This, You Will Understand That…
- AI Facial Recognition works in an automated way using algorithms and deep learning.
- It is a 7-step comprehensive process that creates a digital facial image that can be used as a verified digital identity.
- NIST sets the standards of an ideal facial recognition solution.
- Verification speed impacts the efficiency of a liveness solution in guarding digital identities against spoofing attempts.
- Facia has a state-of-the-art solution testing lab leaving no stone unturned to achieve perfection in identity verification.
Brief on AI Facial Recognition
Artificial Intelligence has been successfully integrated with facial recognition through algorithms and deep learning. AI Face recognition detects and face match patterns that are captured in video or images through high-tech cameras and facial recognition solutions. AI facial recognition involves complex background technical work in various steps. Let us take a look at the working of an AI-based Facial recognition solution.
Steps Involved in AI Facial Recognition
Image Acquisition | Multiple frames of face images are captured using imaging devices.
|
Preprocessing | After image acquisition is completed, preprocessing steps are applied to refine the quality and readability of acquired images. It includes:
|
Facial Feature Extraction | The distinct facial features are extracted from the preprocessed acquired face images. It uniquely represents the human face image correctly identifying all the features. Normally, a facial recognition system extracts three types of facial features:
|
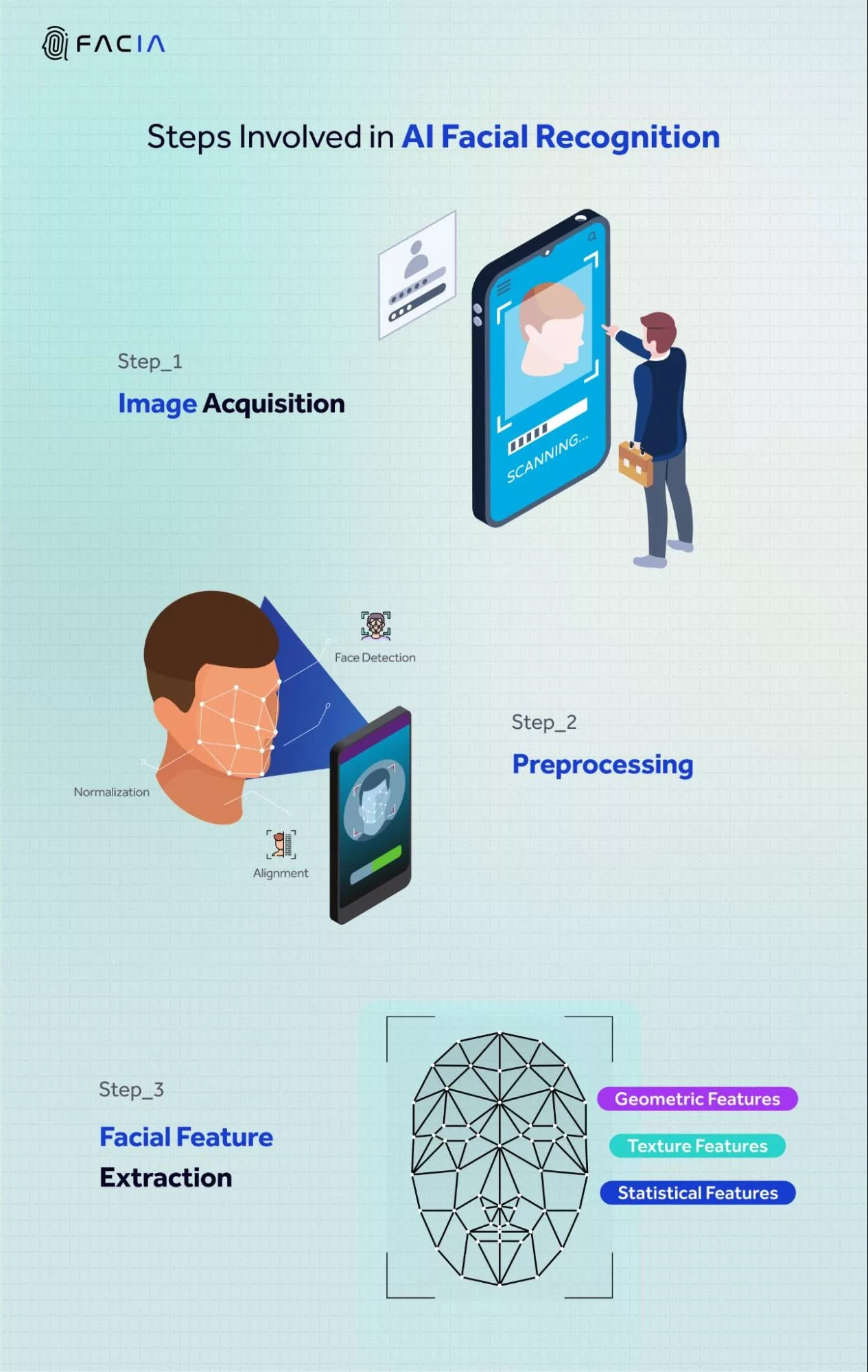
Facial Feature Representation | The extracted facial features are algorithmically and aesthetically combined with sophisticated software to create:
|
Face Database Enrollment & Face Matching | The two feature representations are further worked upon for database matching. The following algorithms execute this step:
Cosine Similarity (A, B) = A . B / ||A||B||
|
Decision Making | The AI face-matching solution makes highly accurate decisions as to whether a face has matched an Identity present in the given database or not.
|
Output | The final output image with a face match score based on the system’s AI-based confidence score is given for the Identity verification decision. |
How AI Facial Recognition Technology’s Accuracy is Measured?
Measuring the accuracy of a Facial recognition solution depends on multiple notions. However, the most effective way to measure the accuracy is to follow the NIST guidelines mentioned in its regularly updated report entitled FRTE (Facial Recognition Technology Evaluation). Recently, the document was republished with updated insights aimed to serve as a benchmark for automated facial recognition technologies.
FRTE evaluated different facial recognition solution providers under four categories of facial image types:
FNMR Category | Testing Algorithm for Image Types | Ideal False Non-Match Rate |
Constrained, Cooperative |
| For VISA and VISA Border = 0.000001 For Others = 0.00001 |
UnConstrained, Non-Cooperative |
| 0.00001 |
How Verification Speed Impacts AI Facial Recognition?
Reaching the benchmark is no less than a challenge especially when it requires meeting the speed goal in verifying Liveness and other aspects of facial recognition. It is to prevent any spoofing attempt to ensure no time window is given to a threat to bypass Identity processing. Speed in verification is aimed at two major goals:
- Preventing identity spoofing threat vectors in less time
- Ensuring seamless user journey.
To achieve this IDV solution testing is carried out in which multiple spoofing attempts are carried out on the solution internally. This helps in improving an IDV solution’s speed and accuracy according to the NIST standards in biometric facial recognition as explained above.
Read More: How To Prevent From AI Face Swap Online Attacks
Few Interesting Insights
According to Gitnux, 71% of retail businesses find facial recognition as a useful security measure. |
iPhone X showed a 0.5% false reject rate in its facial ID feature. This is by far the most accurate facial recognition done through a smartphone. It is because of AI facial recognition technology that Apple uses is uniquely programmed to produce highly accurate results. |
China currently leads the facial recognition technology market having 200 million surveillance cameras having face recognition capabilities. |
How Facia Does Its Testing?
Facia is a cutting-edge facial recognition solution that ensures the highest possible standards in identity verification. Facia’s state-of-the-art testing lab empowers the world’s fastest liveness detection solution by testing it under different conditions and spoof attempts.
The insider’s scoop on liveness verification Buyers Guide
Watch to learn more:
Try Facia and experience the fastest proactive digital identity protection.
Frequently Asked Questions
AI facial recognition is a technology that uses AI facial recognition to identify or verify individuals based on their facial features. It analyzes digital images or video frames to match faces against a database for identification.
It begins with face detection, which identifies faces within an image or video. Then, key features like eyes, nose, and mouth are extracted. The system maps these features into a digital template using mathematical algorithms. This template is then compared with a database to find a match.
Machine learning models, especially deep learning neural networks, are widely used for facial recognition. Convolutional Neural Networks (CNNs) are particularly effective due to their ability to analyze visual data and recognize complex patterns.
Facial recognition has various applications, including:
- Security and Law Enforcement: Identifying suspects or verifying identities at airports and borders.
- Social Media: Tagging people in photos and suggesting connections based on facial recognition.
- Access Control: Unlocking devices or securing buildings through facial verification.
- Retail: Identifying repeat customers or offering personalized recommendations.
While beneficial, AI facial recognition faces some challenges:
- Accuracy Concerns: Facial variations, lighting conditions, and database biases can affect accuracy.
- Privacy Issues: Concerns exist about mass surveillance and potential misuse of facial recognition data.
- Bias and Discrimination: AI algorithms can inherit biases from training data, leading to misidentification.
- Regulation and Ethics: Clear regulations and ethical frameworks are needed for responsible use.