What is False Rejection Rate (FRR)?
Author: teresa_myers | 20 Jun 2024In the previous Knowledge Base, we explained the False Acceptance Rate(FAR) which occurs when a biometric identity verification system falsely accepts a wrong or fraudulent identity. But that is not the only factor affecting the biometric system’s efficiency and accuracy. Another important factor that needs detailed discussion is False Rejection Rate (FRR).
What does FRR Mean?
False Rejection Rate is the frequency at which a biometric identity verification solution rejects a true identity. In other words, the number of false declines by a biometric identity proofing system of a genuine user is called ‘False Rejection Rate (FRR) or False Decline Rate. False Reject Rate occurs when a system doesn’t recognize a genuine user and refuses to give access to a genuine user.
FRR in Biometrics is calculated as:
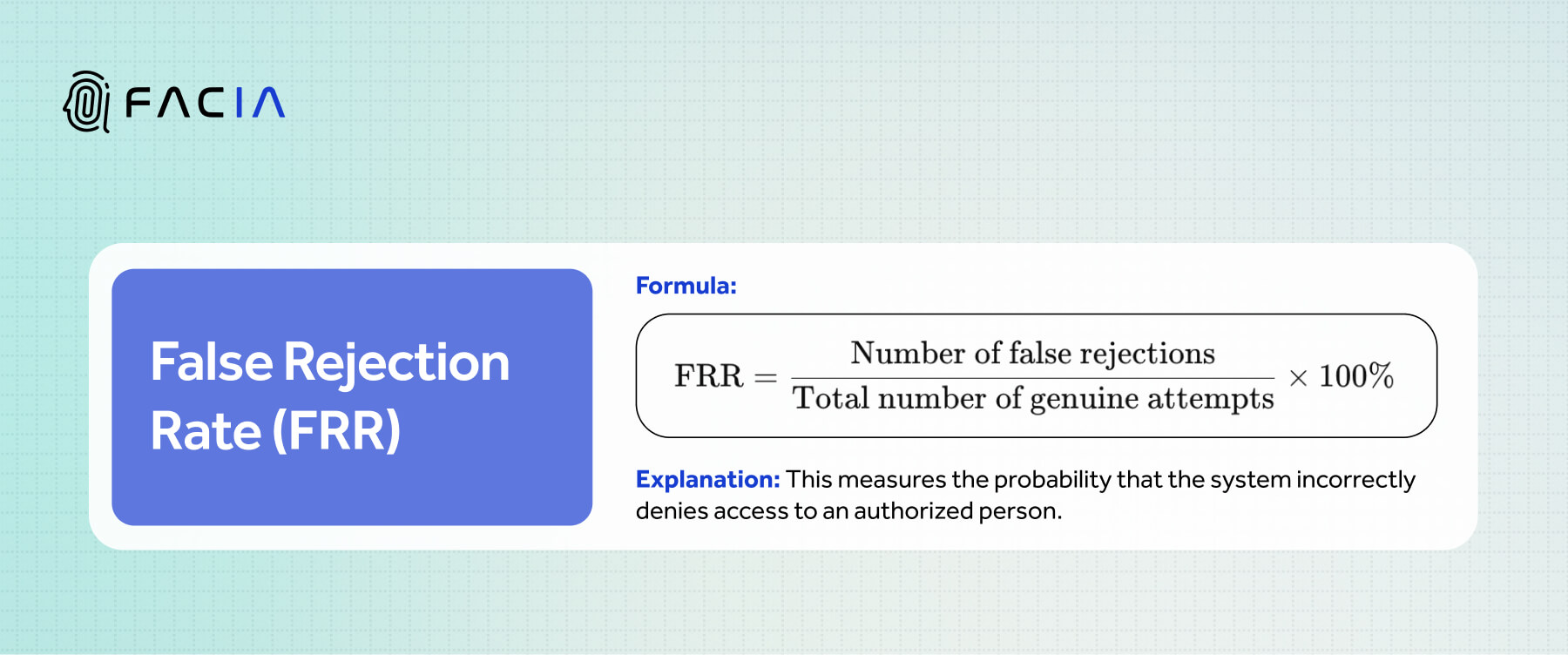
False Rejection Rate itself occurs due to the False Mismatching of biometric identities. A biometric identity verification system let’s say a facial recognition solution can have FRR due to false matching facial identities. Here are the possible reasons for FRR:
- A high False Non-Match Rate (FNMR) is the mismatch of a genuine identity with a false one from the database.
- Incorrect database storage or identity data replacement in the same cell.
- Technical error in the system or facial recognition technology limitation.
Implications of False Rejection Rate
If the identity verification process shows a high level of false rejection rate, there can be multiple problems faced by end-users, businesses, and the biometric identity vendor.
End User |
|
Businesses |
|
Biometric Identity Solutions |
|
False Acceptance Rate (FAR) & False Rejection Rate (FRR)
A general graphical representation of FAR and FRR is given below:
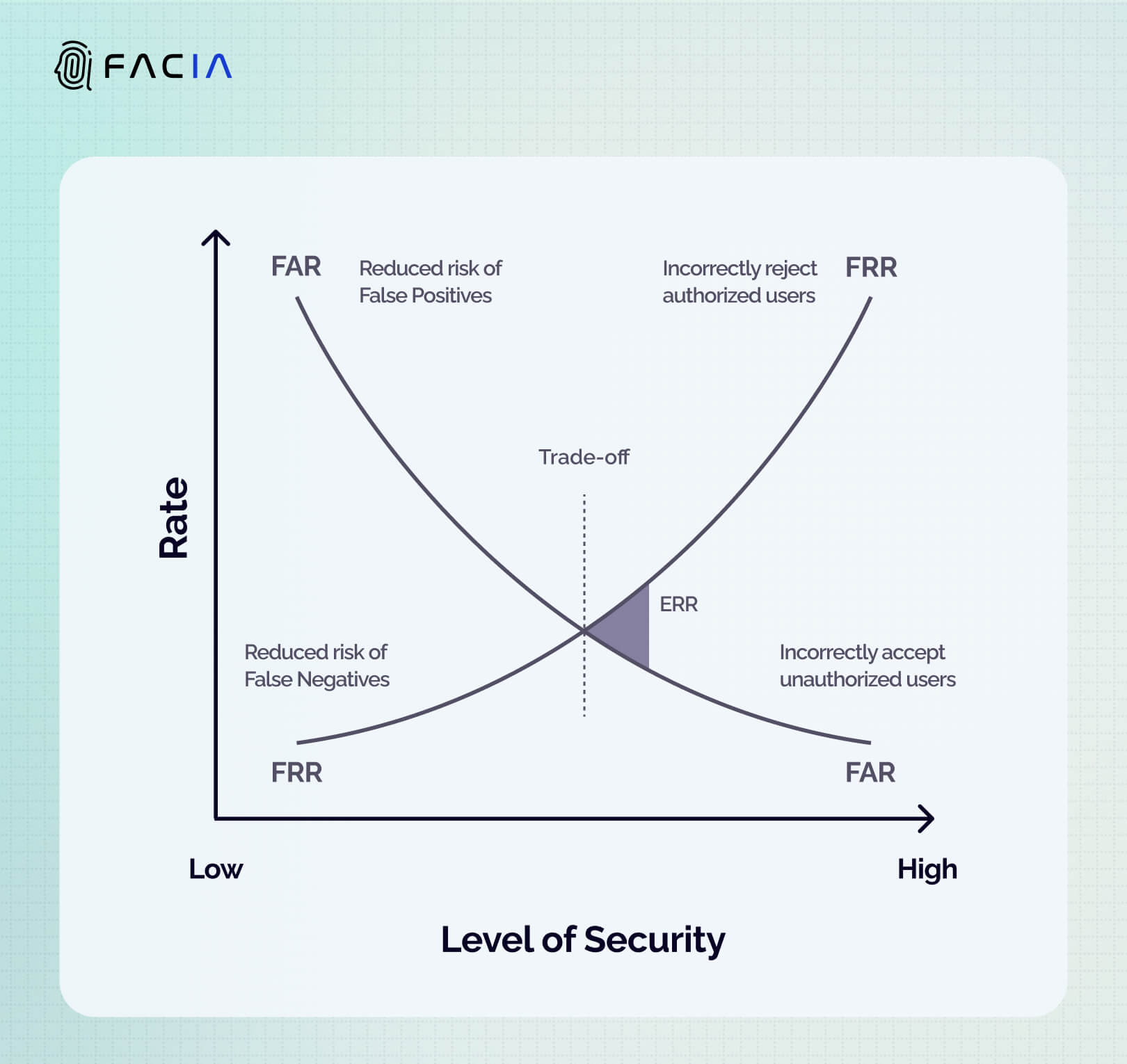
The graph above shows that whenever the False Decline Rate is reduced by a biometric identity solution, it will face an increased False Acceptance Rate where the reducible error will continue to exist.
NIST’s Observation of FRR
According to published research by NIST on 1:N facial identification, here’s a brief overview of NIST setting standards under different settings and input image types for reducing False Negative Identification Rates (also known as False Rejection Rates):
- The change in input image type and number of images presented affected the frequency of FNIR/FRR.
- These FRR rates improved with the diverse image sets provided to the facial recognition system
- It was also found that with a large number of datasets, the FRR rises relatively slower due to the large population size.
- In 1:N matching 12 million facial identities were tabulated for N.
- The element of aging caused False Negatives where changes in facial features and appearance caused time to elapse between the input photographs and the N dataset.
- As Aging is unavoidable and a natural phenomenon, the only solution proposed is to periodically update the databases for the same identities with fresh photographs.
- The ideal standard set in different settings for FRR is 0 to improve user convenience.
- Comparatively, False Negatives appear way less than False Positives in facial recognition algorithms tested by NIST under the demographics category of solution testing.
Tips for Businesses Employing Facial Recognition to Reduce Biometric FRR
- Use high-quality and high-resolution cameras alongside other technologies including systems, etc.
- Optimizing the AI algorithms for facial recognition according to the NIST research and standards can help achieve better results.
- Regular updates in existing customer profiles images, and removing profile duplication in a single database.
- Third-party solution providers should also avoid racial bias in their solutions by enhancing the diversification of facial images to reduce FRR.