What is False Non-Match Rate (FNMR)?
Author: admin | 09 Jul 2024Biometric face recognition is a proactive and highly sensitive tool that requires precision and accuracy at the top to avoid any mismatches in face matching for the identification of users. It is observed that due to the rising numbers of deepfake injection attacks in video calls, and still images for spoofing facial verification process, different error rates become a barrier for FRT in achieving perfection and cause inconvenience to users.
False Non-Match Rate (FNMR) is one of these error rates which will be explained in this knowledge base.
What is FNMR?
False Non-Match Rate is abbreviated as FNMR which is the error rate that occurs in biometric identity verification tools when two identities belonging to the same person are flagged as a non-match.
In simple words, FNMR is an incorrect failure of not recognizing a legitimate user.
It is also known as False Rejection Rate (FRR) by some biometric experts.
Importance of False Non-Match Rate (FNMR)
Understanding and detecting False Non-Match Rates is highly important in Biometric verification systems. Especially when it involves facial recognition service providers, the need for properly detecting and mitigating FNMR becomes crucial because:
- It helps in FRT system evaluation by assessing its performance under different conditions and exposures.
- It helps in maintaining an optimal balance between user convenience and security levels.
- It helps in pinpointing the system’s weaknesses such as a loophole in the algorithm, technological limitations, etc.
False Non-Match Rate Formula
False Non-Match Rate (FNMR) is calculated as follows:
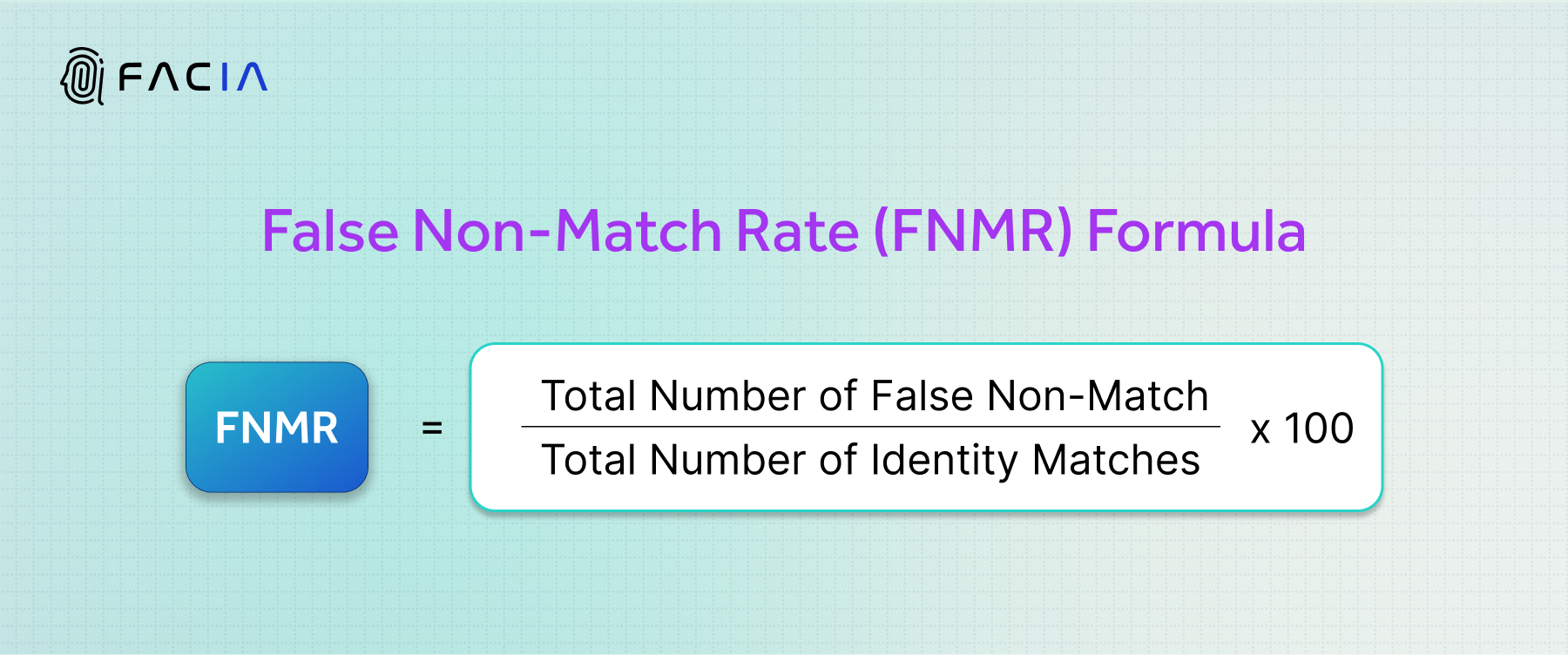
Suppose a facial recognition system incorrectly rejects 3 users out of 100 faces presented to the camera, its FNMR will be 3%.
Impact of False Non-Match Rate (FNMR)
The biggest risk of having FNMR is the loss of genuine customers due to inconvenience in identification. If a facial identity proofing system repeatedly rejects a genuine user’s face by not recognizing him or mismatching the face with a potential threat, it will cause frustration and users may quit using the service.
Other impacts of False Non-Match Rate include:
- Decreased efficiency of the Facial Recognition Tool
- Increase in cost of threat prevention and error rate correction
- Hurts the overall brand worth.
FMR vs. FNMR | Reaching the Balance through Trade-off
It is a fact that No system made by man is 100% perfect. The same is the case with facial recognition solutions. The opposite of a False Non-Match Rate is the False Match Rate (FMR) which is the incorrect recognition of a facial identity as a legitimate user.
Researchers have tested several facial recognition tools and their algorithms in an attempt to reach 100% accuracy and reduce both FMR and FNMR to zero yet it is impossible due to the trade-off between both. It means that trying to reduce FNMR would increase FMR and vice versa.
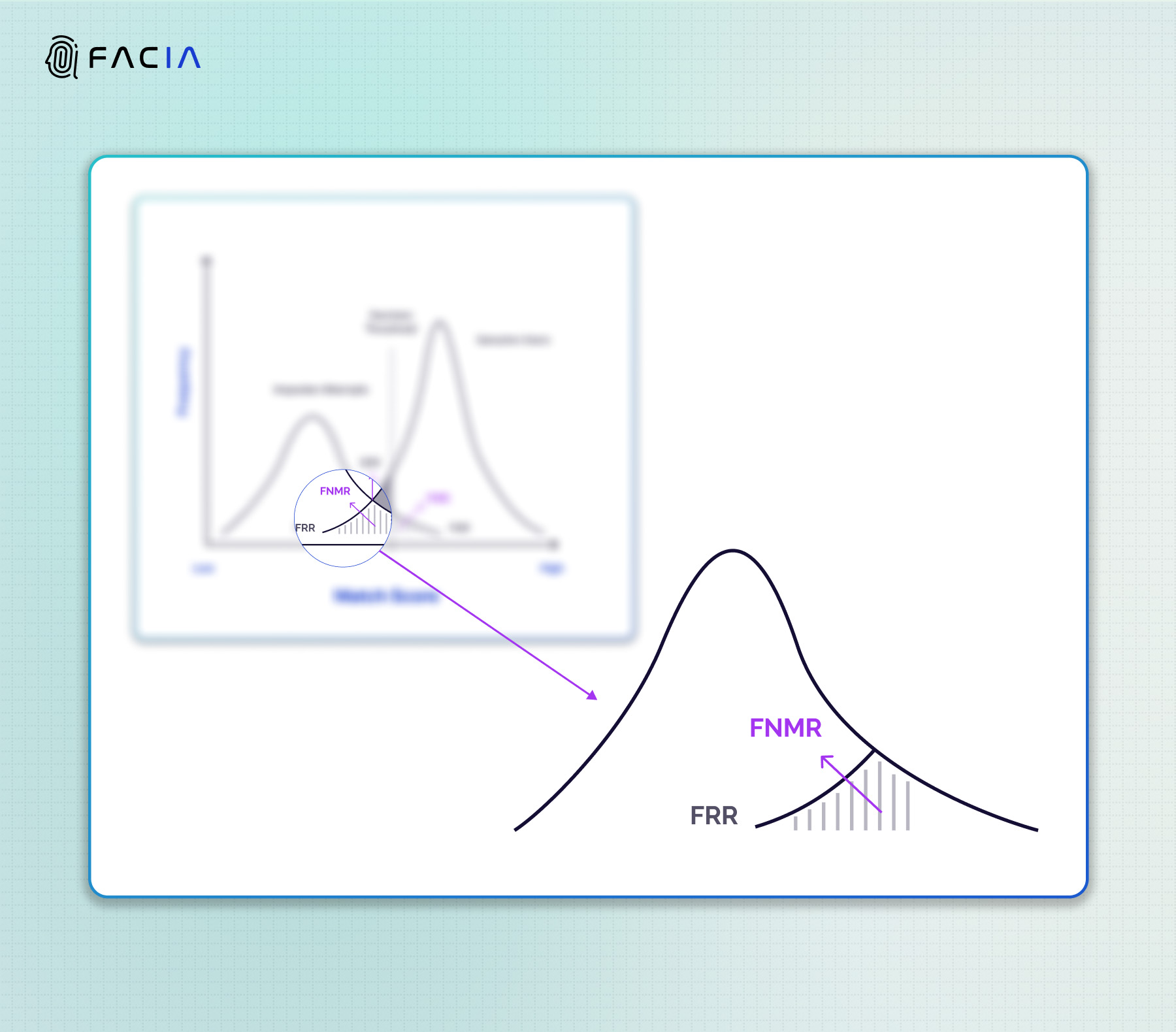
It is highly important to set the threshold of FNMR and FMR at the optimal levels keeping in view the facial recognition tool’s actual ability, cost constraints, and user convenience.
Factors Affecting False Non-Match Rate (FNMR)
1. Image Quality
Facial images taken from a high-resolution camera and at an appropriate angle will help reduce the False Non-Match Rate.
2. Lighting Condition
Proper lighting and exposure to face highly impact the FNMR rates. For example light at the back of the head may increase in False rejection.
3. Facial Expression
It may affect the FNMR rates in older FRTs but in the latest AI-powered facial recognition solutions, facial expressions are also detected and interpreted while conducting face matching. But there may remain a slight room for error like FNMR in matching extreme facial expressions.
4. Occlusions
Occlusions are obstructions in the way of recognizing a facial image or another biometric trait. In facial identity verification, facial occlusions are the objects, wearable gear, and artifacts that can block the view of an FRT camera, and the face may not be recognized at all. Facial occlusions include glasses, hats, mustaches & beards, masks, etc.
These are also called partial facial occlusions.
5. Algorithm Robustness
It marks the ability to accurately identify and detect a user’s image through trained AI and ML models.
6. Database Size
The larger the database, the higher the chances of a False Non-Match Rate (FNMR) to occur.
How False Non-Match Rate (FNMR) can be Reduced or Prevented?
First and the foremost step is to use the right equipment, environment, and settings considering the vital factor of user convenience. Legitimate users will find it helpful and convenient if the FRT application software has proper runtime instructions and pop-ups in easy to understanding manner steering the user to properly use FRT through mobile phones as FNMR is highly likely to appear more in remote settings like mobile phone selfie-based verifications. Despite this, the reduction of the False Non-Match Rate may remain a challenge guiding every mobile phone user regardless of factors like age, tech-savviness, etc.
However, in highly sensitive and secure uses like border control or law enforcement use cases the high FNMR levels can’t be tolerated.