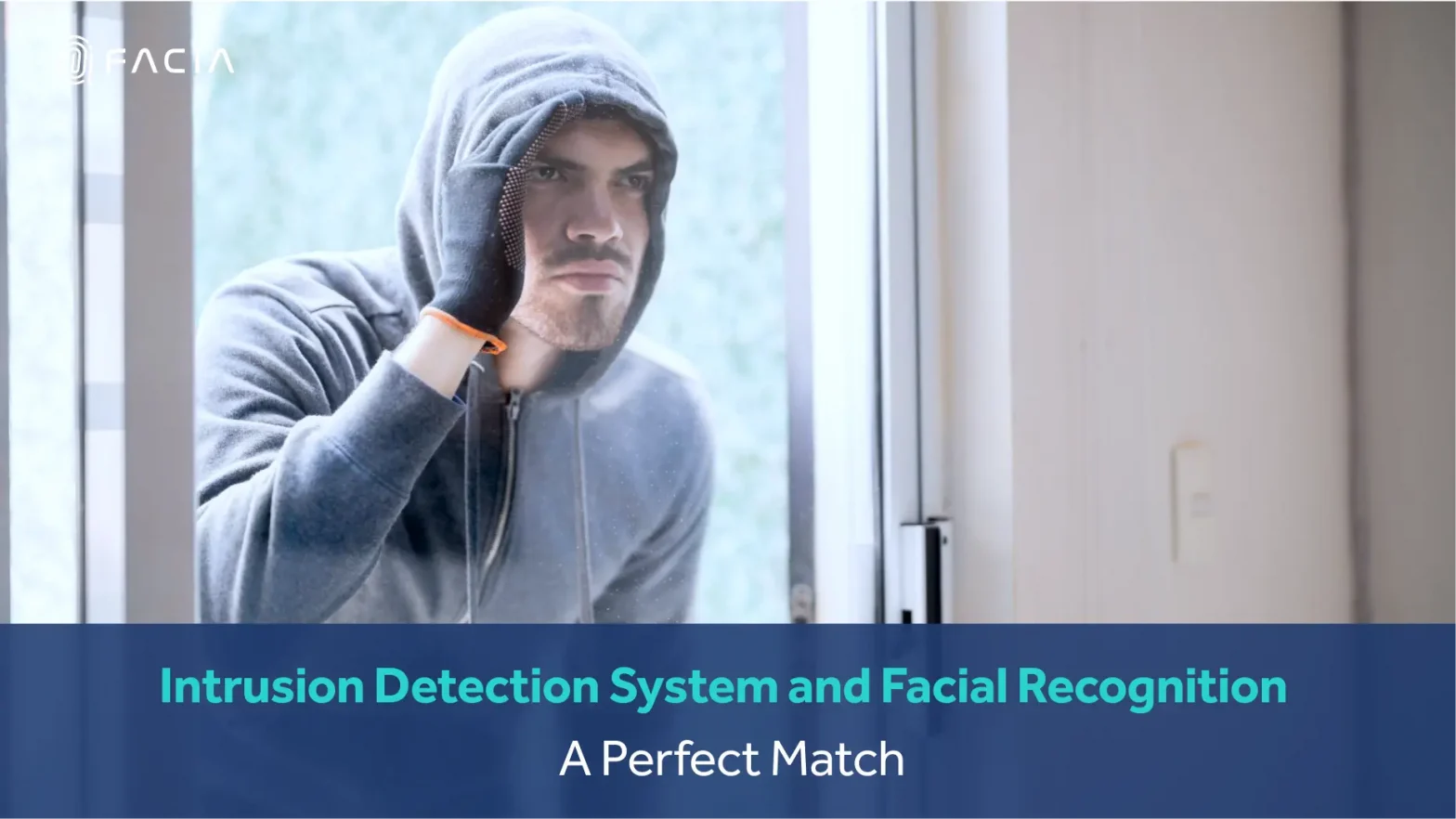
Intrusion Detection System and Facial Recognition | A Perfect Match
Author: admin | 01 Aug 2024In This Post
What’s the best way to stop an intruder before entering your premises? It is to identify an intruder outside the premises and report him right away to law enforcement. To do it in a frictionless manner, intrusion detection systems must be equipped with identity verification technology that is unconstrained, handy, speedy, and accurate. We prefer saying that biometrics like facial recognition is the best tool for intrusion prevention in multiple environments.
Let’s shed some light on using Face Recognition for Intrusion detection & prevention systems in today’s blog.
Intrusion Detection System Overview
Generally, an Intrusion Detection System (IDS) is a security solution designed to detect unauthorized access and physical breaches within protected premises. Facial recognition technology is leveraged to enhance intrusion detection and prevention by recognizing and detecting the faces of intruders and differentiating them from allowed personnel in a monitored area.
Intrusion doesn’t only mean a physical unauthorized entry into a building or premises. Intrusion can also occur digitally where cybercriminals bypass the security barriers and break into restricted cyberspaces for many illicit gains:
- Information and data theft
- Identity theft and identity fraud
- Cryptocurrency fraud
Facial recognition tool in an intrusion prevention system will carry out a 1 to 1 and 1 to N face matching of a person whose face appears in front of an embedded device having a smart camera.
The comparison is carried out as:
- 1 to 1 against the individual’s identifiable information present in the system. It is to verify that a person is the actual owner of the identity presented to the facial recognition tool.
- 1 to N face matching against a database of people who are authorized to enter the monitored premises.
If an identity doesn’t satisfy either of the above face-matching procedures, the system takes action against the individual by reporting the individual or alerting the security personnel to stop him.
Deep Learning | The Catalyst of Successful AI Intrusion Detection System
Deep learning is a branch of Machine Learning that allows the use of neural networks (in this case Convolutional Neural Networks), composed of abundant layers that train a system by learning from represented input data at an increased level of abstraction in each layer. Security Intrusion Detection Systems have benefitted from Deep Learning models in the following ways:
- Near-human level accuracy in detecting intrusion. Approximately 99% accuracy in detecting labeled faces.
- Facial image classification and recognition in the given dataset.
- Occlusion detection helps in detecting faces that try to cover behind an object to spoof a facial recognition camera.
- Gait recognition also helps in human behavior analysis and prevents intrusion by recognizing a person’s walking style.
- Sentiment analysis helps in predicting a human being’s actions shortly.
- Gaze Tracking helps in predicting human intentions and behavior.
Types of Intrusion Detection System
1. Signature-Based Intrusion Detection System (SIDS)
Signature-based IDS works on known patterns of intrusion. It is a knowledge-based system that works with the matching methods and detects previous intrusion attempts. Simply, when an intrusion signature matches with a previously detected intrusion signature, the system raises the alarm.
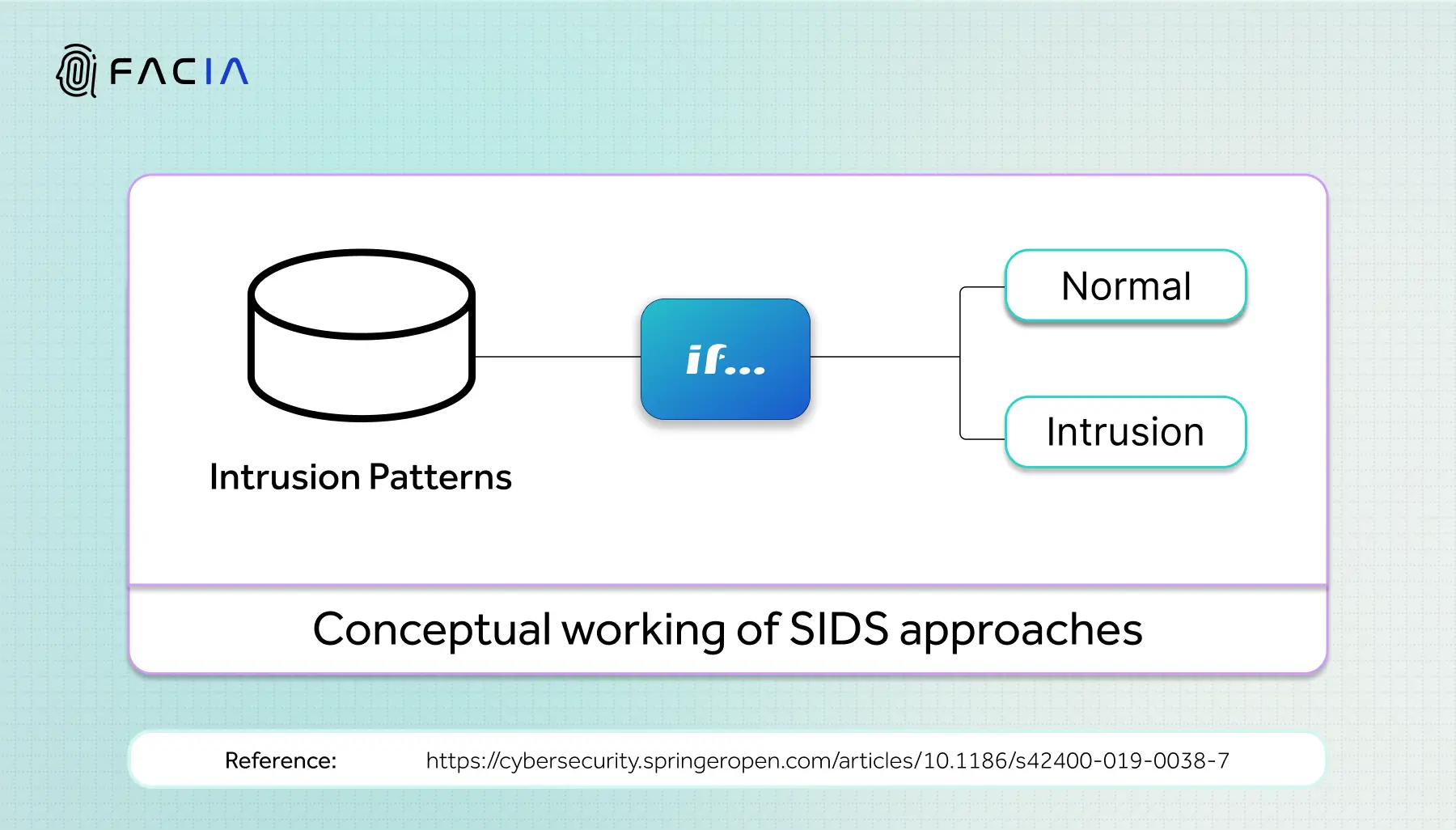
2. Anomaly-Based Intrusion Detection System (AIDS)
Anomaly-based IDS (AIDS) is aimed at overcoming the loopholes in SIDS. It employs machine learning, knowledge-based, and statistical analysis methods. A significant deviation noted between the observed behavior and the model will be detected as an anomaly. This anomaly will be interpreted as an intrusion. It further involves 2 steps:
- Training phase in which normal profiles are presented to the facial recognition system for training the ML model.
- Testing phase in which a new dataset having anomalies is presented to the system. It generalizes the preciously undetected intrusions.
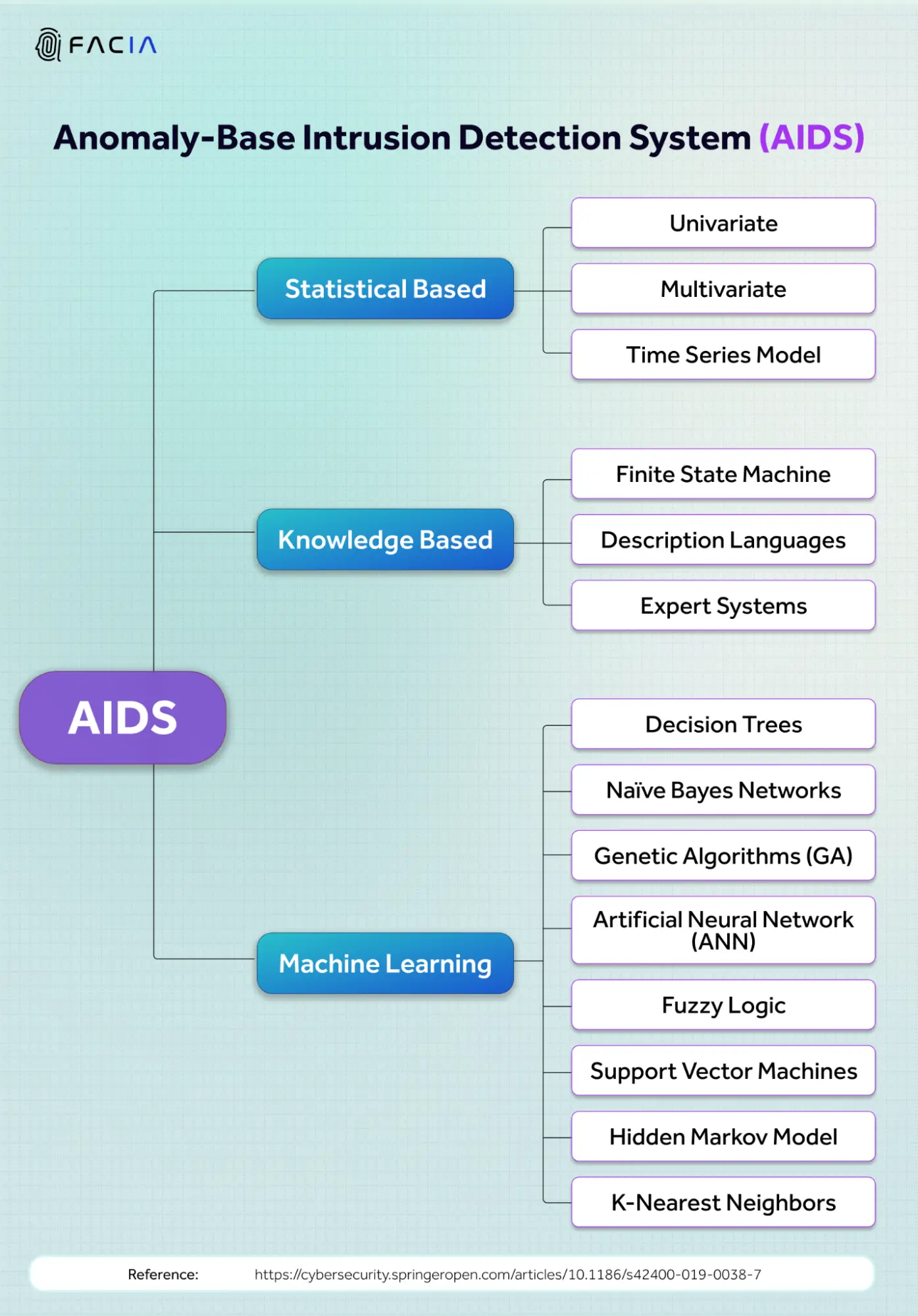
Performance Metrics for Intrusion Detection System
Just like other biometric authentication tools, intrusion detection systems using facial recognition are evaluated based on the following standard performance measures:
1. False Positive Rate (FPR)
The threshold with which an IDS incorrectly authorizes an intruder or fails to detect an intrusion attempt.
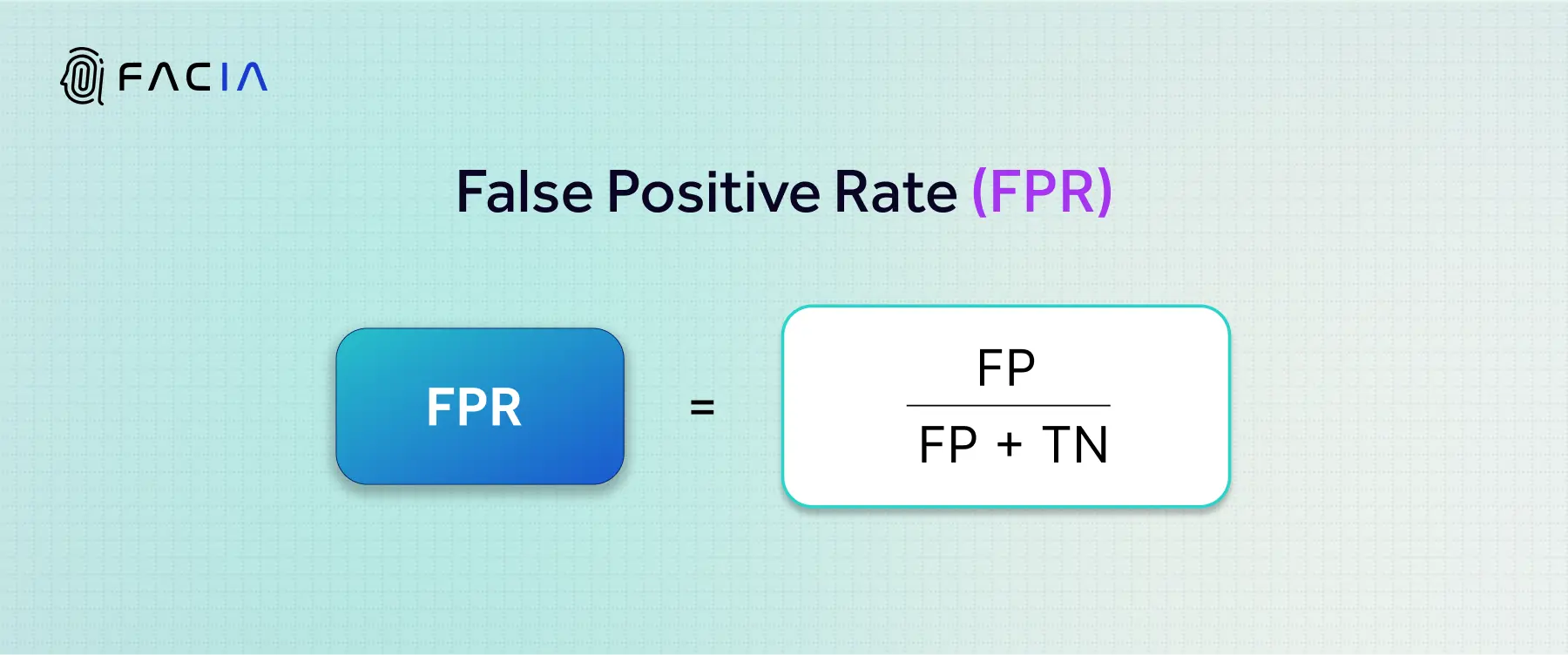
2. False Negative Rate (FNR)
It is the error rate with which an Intrusion Detection System falsely identifies a true user as an intruder.
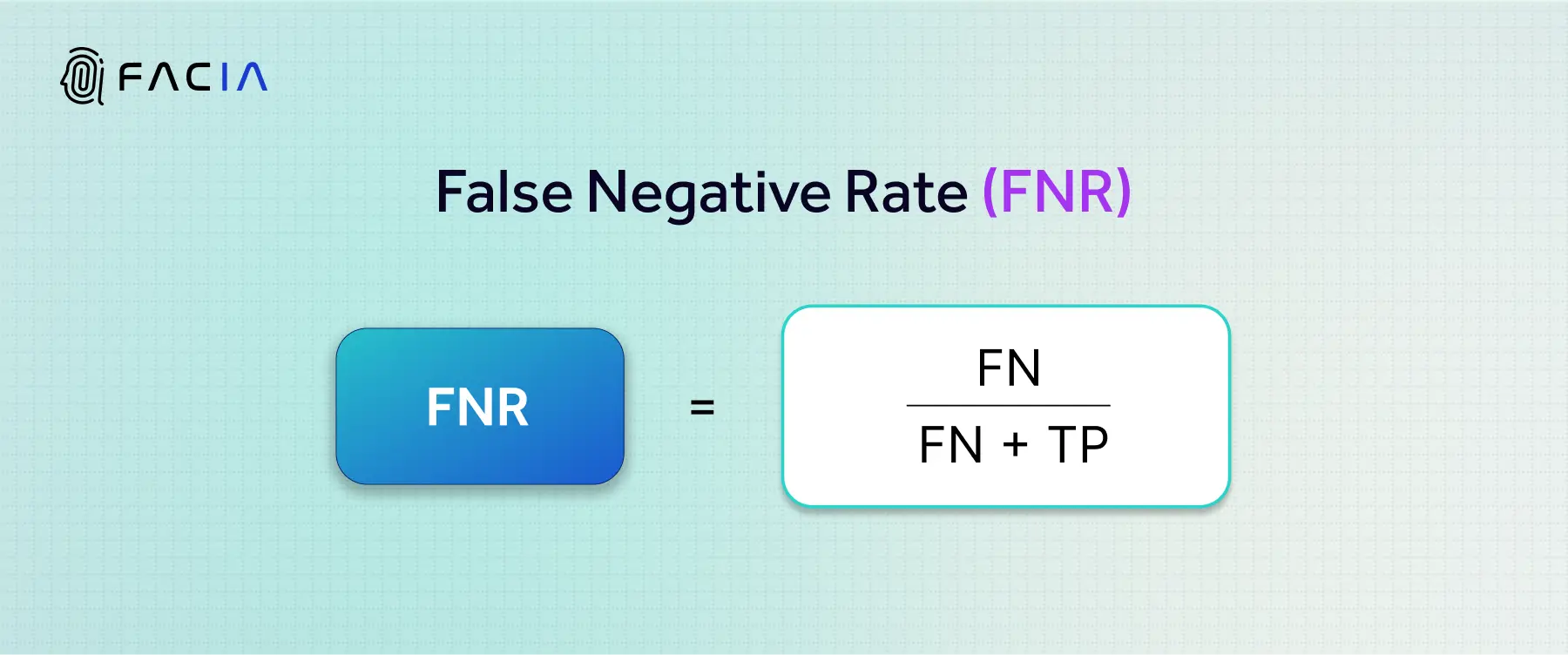
3. True Positive Rate (TPR)
It is the number of correct authentication of genuine users by an IDS using facial biometrics.
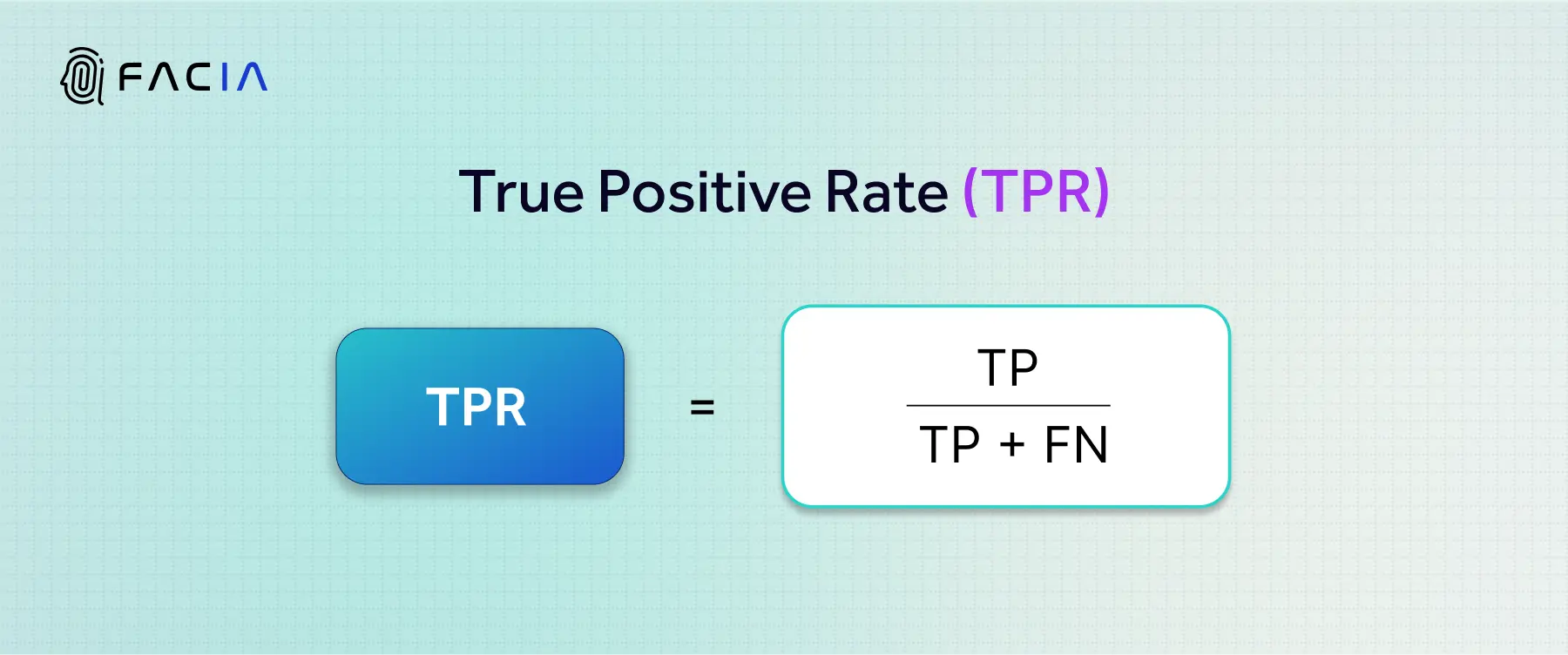
4. True Negative Rate (TNR)
It is the number of correct detection intrusion attempts by an Intrusion Detection System.
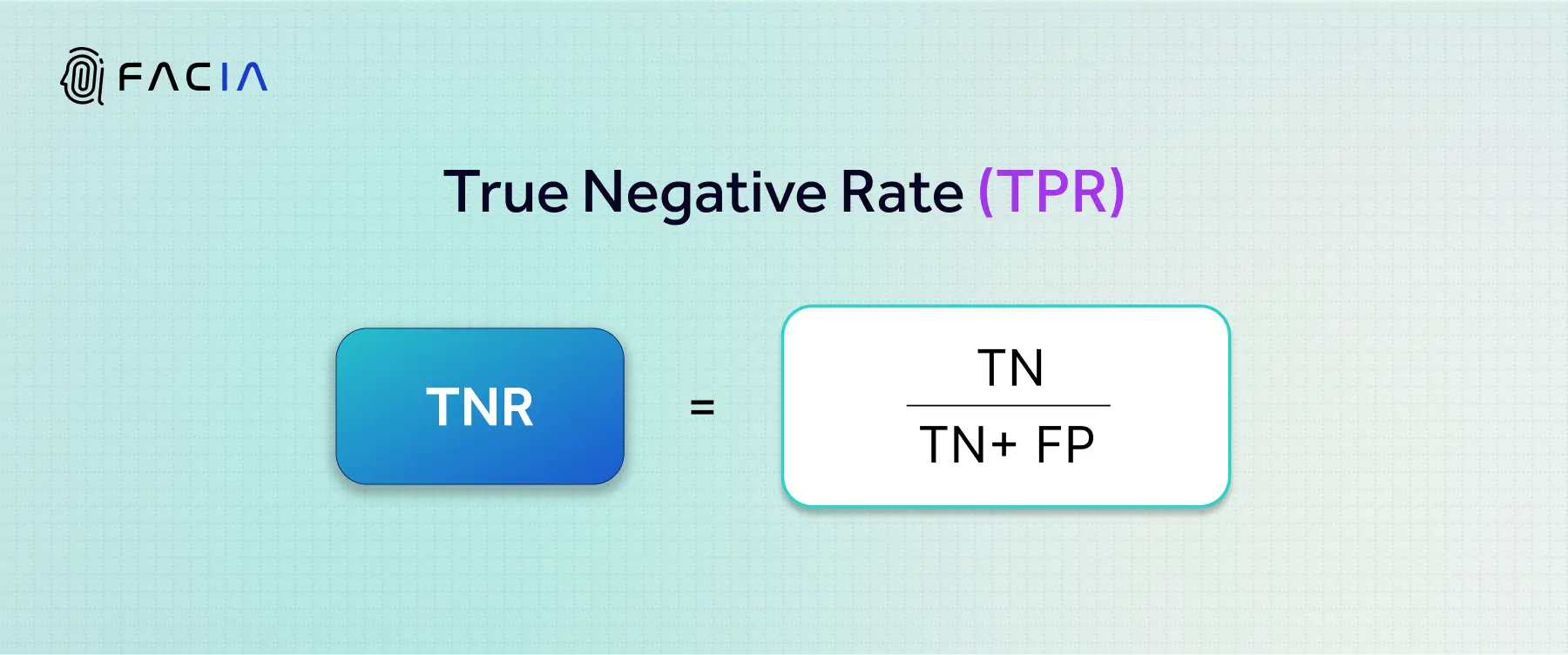
5. Accuracy or Classification Rate (CR)
It is the percentage of all correctly detected intruders and users to all the presented attempts. It measures the accuracy level of an intrusion detection system.
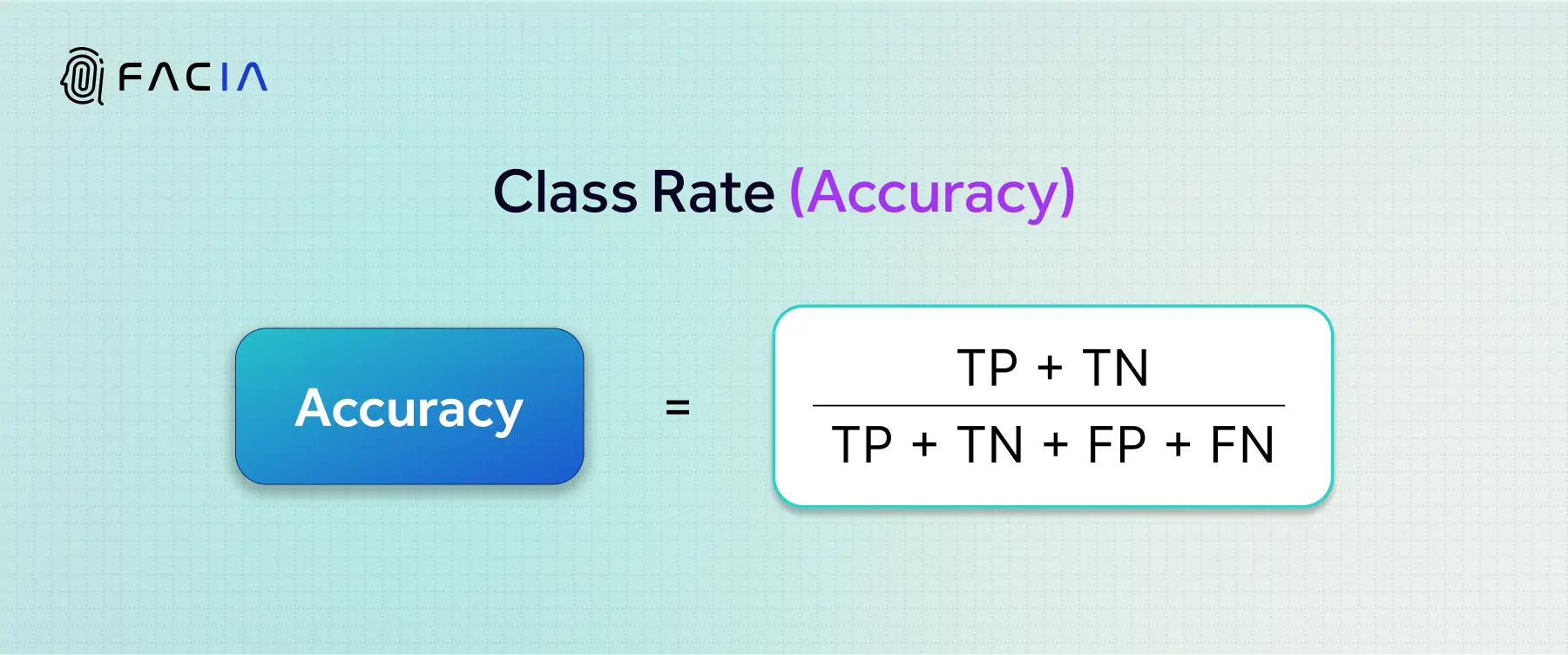
Deepfakes | Outsmarting Intrusion Detection System and Face Recognition
A report pinned the evolution of malicious software as the critical challenge intrusion detection systems (IDS) faced. Malicious attacks refer to the creation of highlight sophisticated and difficult-to-detect tactics to bypass intrusion detection in all settings. Pertinent to Facial recognition the creation of deepfake images and deepfake injection attacks have heightened the threat levels.
A deepfake is a highly convincing and realistic-looking picture, voice, or video manipulation technique that can confuse and outwit the biometric authentication used in intrusion prevention systems. Recently, deepfakes have been observed as one of the most threatening malicious attacks in the cyber world.
Liveness Detection | A Perfect Firewall Against Intruders
AI intrusion detection incorporates Liveness detection for enhanced intrusion detection and prevention. Facial recognition technology used for intrusion detection requires robust methods to detect anomalies and different malicious attacks such as deepfakes as discussed earlier. To detect and prevent these, an early liveness check will ensure that the person trying to enter cyberspace is a genuine permitted user and not a deepfake intrusion attack.
Facial recognition tools like Facia offer AI Liveness Detection to fortify the intrusion prevention system by detecting deepfakes and other spoofing attempts. It is a regularly updated liveness verification program that will give no breathing space to any intruder online and on-premises. Secure your buildings, online spaces, and beyond with Facia against intruders.
Bottomline
An intrusion detection system highly depends upon the robustness of the facial recognition solution deployed at the heart of identity verification to improve accuracy and speed of detection. Liveness plays a critical role in detecting cyber intrusion particularly the ones using deepfakes and other facial identity spoofing tactics. Opting for a robust liveness-based facial recognition tool will sow the seed of continuous improvement and prevent intrusion attempts in real-time.
Frequently Asked Questions
Facial recognition plays a critical role in intrusion detection systems as it recognizes the faces of intruders and helps in accurately identifying authorized users and potentially threatening personnel.
Integrating facial recognition technology with an intrusion detection system enhances the overall security as accurately detecting faces is critical for the firm’s on-premises and online security. It does it by ensuring that no spoofing attack is carried out or no dangerous person has entered the space without going undetected. Once a face is recognized as an intruder the firm can take swift actions to stop the harmful activities.
Traditional methods of intrusion detection such as hiring security guards who personally keep a watch have loopholes like missing out on a random intruder or not having a clear view of the faces. They might even miss out on a possible mask attack or even they can easily be overpowered by an armed intruder if not properly equipped. Similarly, traditional intrusion detection in cybersecurity is also limited to carrying out simple security checks like security questions or a password. Facial recognition resolves both ends of the problem by offering liveness detection for accurately recognizing the faces of the individuals and reporting them.