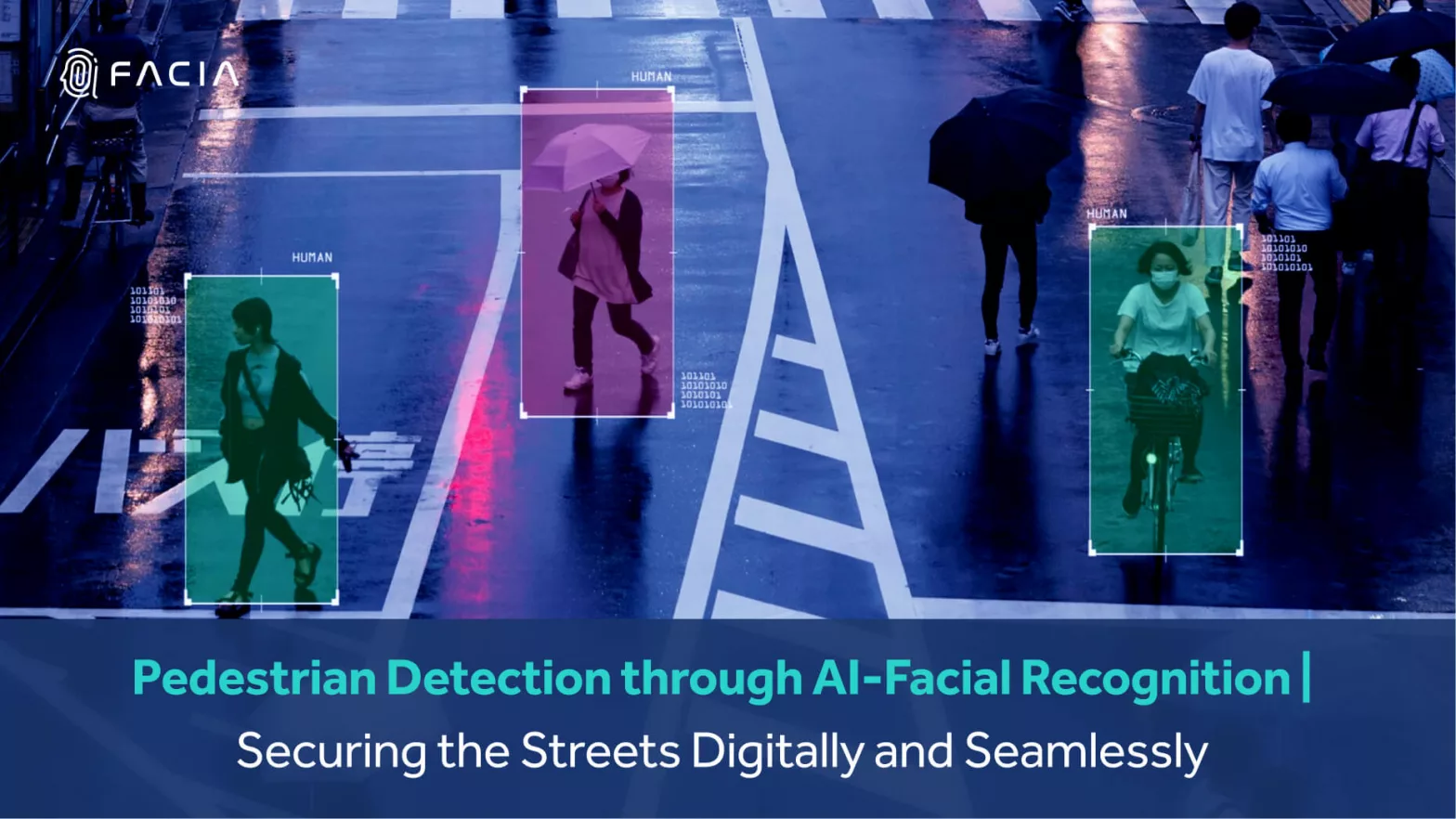
Pedestrian Detection through AI-Facial Recognition: Securing Crowds with AI
Author: admin | 08 Apr 2024In This Post
Imagine a fraudster escaping a bank right after his latest heist and tactfully vanishing in the crowded pedestrian lanes outside the bank posing a risk to the safety of the public due to being unidentified. Owing to rapidly evolving economic landscape, people prefer to actively participate in activities conducted in public areas. Crowded places like football stadiums, concert halls, shopping malls, etc act as a safe hideout and escape zones for criminals. To achieve global perfection in compliance through crime prevention, pedestrian detection through advanced facial recognition techniques is attracting attention.
In this blog, we will analyze the existing pedestrian detection systems, categorize them and we will attempt to relate how facial recognition systems can enhance crime prevention by detecting pedestrians.
Key Takeaways
- Facial recognition improves pedestrian detection accuracy, especially in crowded areas.
- Facial recognition integrates seamlessly with existing pedestrian detection technologies for a robust security system.
- Facia can perform 1:N matching against a large image database in under 4 seconds, making it ideal for real-time pedestrian detection scenarios where swift identification is crucial for security personnel.
- Liveness detection, camera quality, and user consent are crucial factors for successful facial recognition implementation.
What is Pedestrian Detection?
Pedestrian Detection is a digitally operated identification system used to identify pedestrians and ensure public safety. It is considered a vital part of crowd surveillance and identifying suspicious individuals. Most pedestrian detection systems use facial recognition methods of some sort. But with advancements like AI, deepfakes and other identity fraud practices are becoming increasingly common. Thus, to mitigate this threat, advanced facial recognition systems are drawing every concerned party’s attention and interest to enhance pedestrian recognition systems.
Categories of Pedestrian Detection System
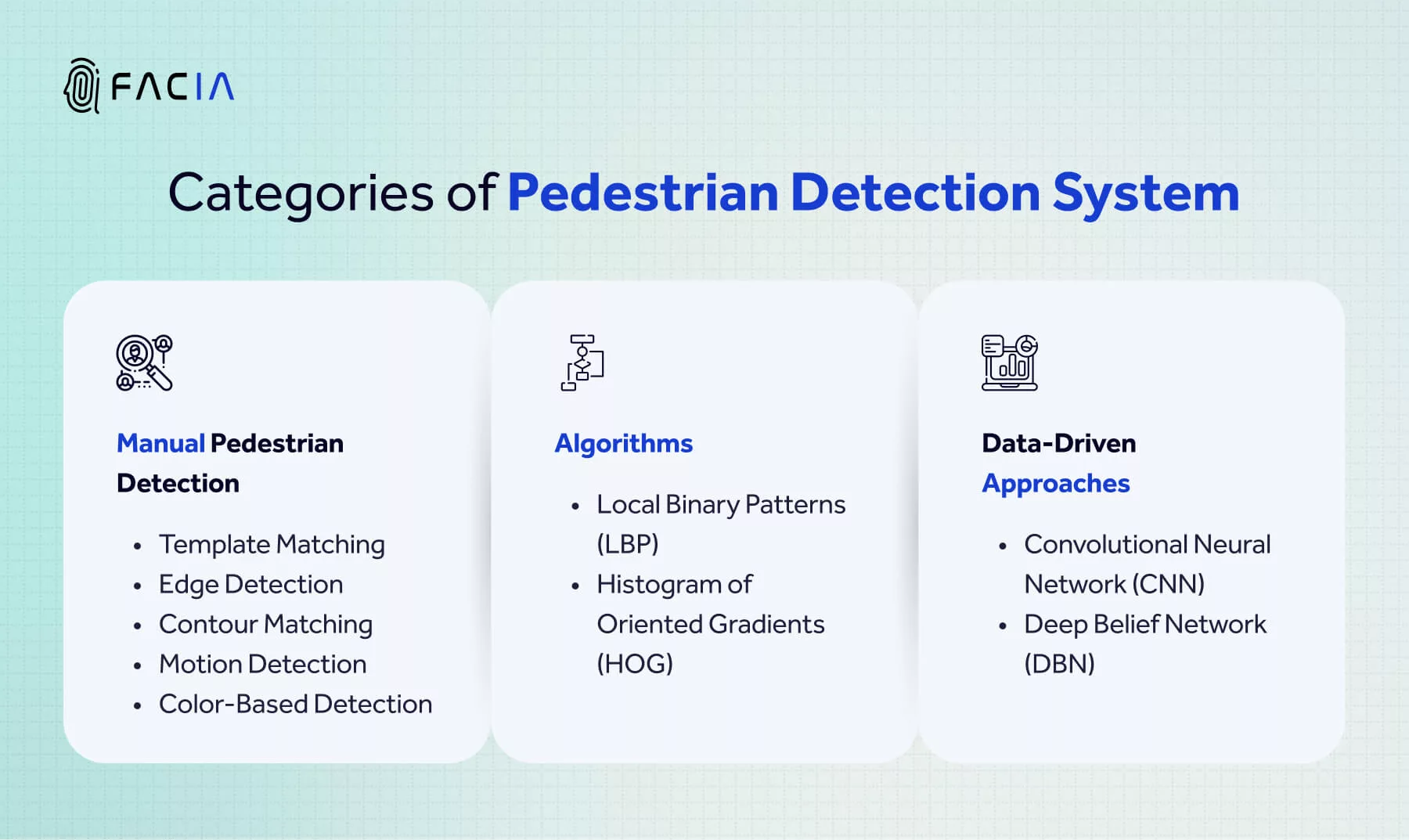
Manual Pedestrian Detection
Manual pedestrian detection uses visual inspection techniques for detecting individuals in crowded places. This includes:
- Template Matching incorporates predefined templates and matches the regions with images or video recordings of CCTV cameras.
- Edge Detection identifies pedestrian body edges and silhouettes captured in the image using canny edge or other techniques. This helps detect only the number of pedestrians but cannot recognize a facial identity.
- Contour Detection brings in the element of boundaries and contour of pedestrians with changing intensity in the image recorded.
- Motion Detection reads the human gestural movements, gate, posture, and other motion factors on pedestrians by reading the video sequences.
- Colour-based detection detects pedestrians based on skin colour and physical appearance in the image.
Algorithms
Algorithms help in automating the identity verification process. For pedestrian detection, there are 2 major approaches including:
- Local Binary Patterns (LBP) is an image processing algorithmic technique that works by comparing pixels with neighbouring pixels that create a binary pattern depending on the color brightness of the neighbouring pixel. This pattern describes the texture of the image captured.
- Histogram of Oriented Gradients (HOGs) is another computer vision and image processing technique whose algorithm detects an object from an image. It divides an image into smaller regions, calculates the gradient direction and magnitude of each region, and then builds a histogram of gradient orientation. It uses machine learning algorithms mostly.
Data-Driven Approach
- Convolutional Neural Networks (CNN) is an effective image recognition and classification technique. It uses image layering to automatically and adaptively learn spatial hierarchies of features from the input images. Convolutional Layers, pooling layers, and fully connected layers are used in the CNN approach for image recognition.
- Deep Belief Network (DBN) is a generative neural network technique that works on multiple hidden layers and a visible layer in the image. The image recorded in DBN is composed of layers from Restricted Boltzmann Machines (RBMs). The DBN is trained in an unsupervised manner using techniques like contrastive divergence. It is used for feature learning, dimensionality reduction, and unsupervised pre-training of deep neural networks.
How Facial Recognition Technology Enhances Pedestrian Detection System?
Facial Identity Verification lies at the forefront of digital identities. Pedestrian detection for ensuring public safety requires swift and accurate detection of suspicious actors to respond to a possible threat promptly. For this purpose, facial recognition technology can enhance pedestrian detection in several ways:
Pedestrian detection can be done with higher accuracy and speed through advanced facial recognition technology. |
Facial recognition can be combined with the technologies discussed above to create a multi-modal robust pedestrian detection system. |
Facial recognition helps in identifying unique identifiers in crowded places for all individuals in the coverage area. |
Face recognition can also provide contextual information about identified pedestrians (age, gender, emotional state, etc.) |
It can fortify the fight against crimes by timely identifying and reporting criminals mixed up in a pedestrian lane. |
Key Considerations for Implementing Facial Recognition in Pedestrian Detection
Multiple factors are the core determinants of scaling the performance of a face identity verification solution. Look at the table below to understand how facial recognition impacts user identification for single and multiple-user settings:
Single-User Settings | Multiple-User Settings |
|
|
Apart from the above considerations, several factors impact both single & multiple user facial verification such as:
- Liveness Detection ensures that no single or multiple user is using a mask attack or deepfake injection attack for identity spoofing. Facial Recognition solutions must be able to detect the latest identity spoofing techniques through AI and Machine learning.
- The camera’s distance and resolution in pedestrian detection and how quickly identities can be verified are highly important. The IDV solution must incorporate high-end devices and systems to expand the scope and speed of facial identity verification.
Facia – A Solution for Fast and Accurate Pedestrian Detection
Facia helps businesses and organizations vigilantly identify any risky profile anywhere. With the ability to detect liveness in facial recognition in under one second, Facia is a certified identity solution that has multiple use cases. For Pedestrian detection, Facia can detect liveness and verify an entire crowd’s identities, ensuring high speed and accuracy.
Final Word
Pedestrian Detection systems lie at the core of public safety. Government agencies and regulators should primarily focus on enhancing pedestrian identification through advancements in the field of AI and machine learning. For this purpose, facial recognition solutions are advised to enhance their solutions against the rapidly evolving threat vectors in facial identity spoofing.
Frequently Asked Questions
A pedestrian detection system is a technology that uses cameras and sensors to identify people on foot in an image or video stream. This is important for various applications, including:
- Public safety: Helping to monitor crowds and identify suspicious activities.
- Traffic management: Detecting pedestrians near roadways to prevent accidents.
- Retail analytics: Understanding customer behavior in stores.
Pedestrian detection employs computer vision algorithms to analyze video footage or images. These algorithms look for specific patterns and features that are characteristic of pedestrians. Steps in pedestrian detection:
- Background subtraction: Establish a background reference to differentiate moving objects (pedestrians) from static elements.
- Motion detection: Identify areas of movement within the image or video frame.
- Shape and size analysis: Analyze the shapes and sizes of moving objects to identify pedestrian-like proportions.
- Classification: Classify the detected object as a pedestrian based on extracted features like motion, shape, and size.
Pedestrian recognition is a more advanced technology that builds upon pedestrian detection. It aims to not only identify the presence of pedestrians but also potentially recognize individuals. This can be achieved through techniques like:
- Facial recognition: Matching faces against a database (1:N) to identify specific people.
- Gait analysis: Identifying individuals based on their walking patterns.
- Clothing recognition: Identifying people based on what they are wearing (less common).
Artificial intelligence (AI) plays a crucial role in pedestrian recognition, particularly with facial recognition. AI algorithms can:
- Analyze video footage and identify human forms.
- Extract facial features from images.
- Compare those features to a database of known individuals.
- Continuously learn and improve their accuracy over time.
Facial recognition improves the accuracy of pedestrian detection, especially in crowded areas. Traditional methods may struggle to differentiate between people, whereas facial recognition can pinpoint and find individuals(1:N idenifiication) with greater precision. However, accuracy can be affected by factors like:
- Camera quality: High-resolution cameras are necessary for accurate facial recognition.
- Lighting conditions: Poor lighting can make it harder to capture clear facial details.
- Facial variations: Angles, occlusions (like sunglasses), and facial expressions can affect recognition.
Facia's advanced facial recognition system empowers pedestrian recognition with a robust feature set. This includes 1:1 and 1:N identification, image-to-video matching, and even video-to-video identification. Additionally, Facia can estimate age and recognize emotions, providing valuable contextual information. These features, coupled with Facia's high accuracy and processing speeds under 4 seconds, enable efficient identification of individuals in real-time and across diverse environments. This swift and precise recognition is crucial for pedestrian detection systems, allowing them to flag potential threats and alert drivers or security personnel promptly.