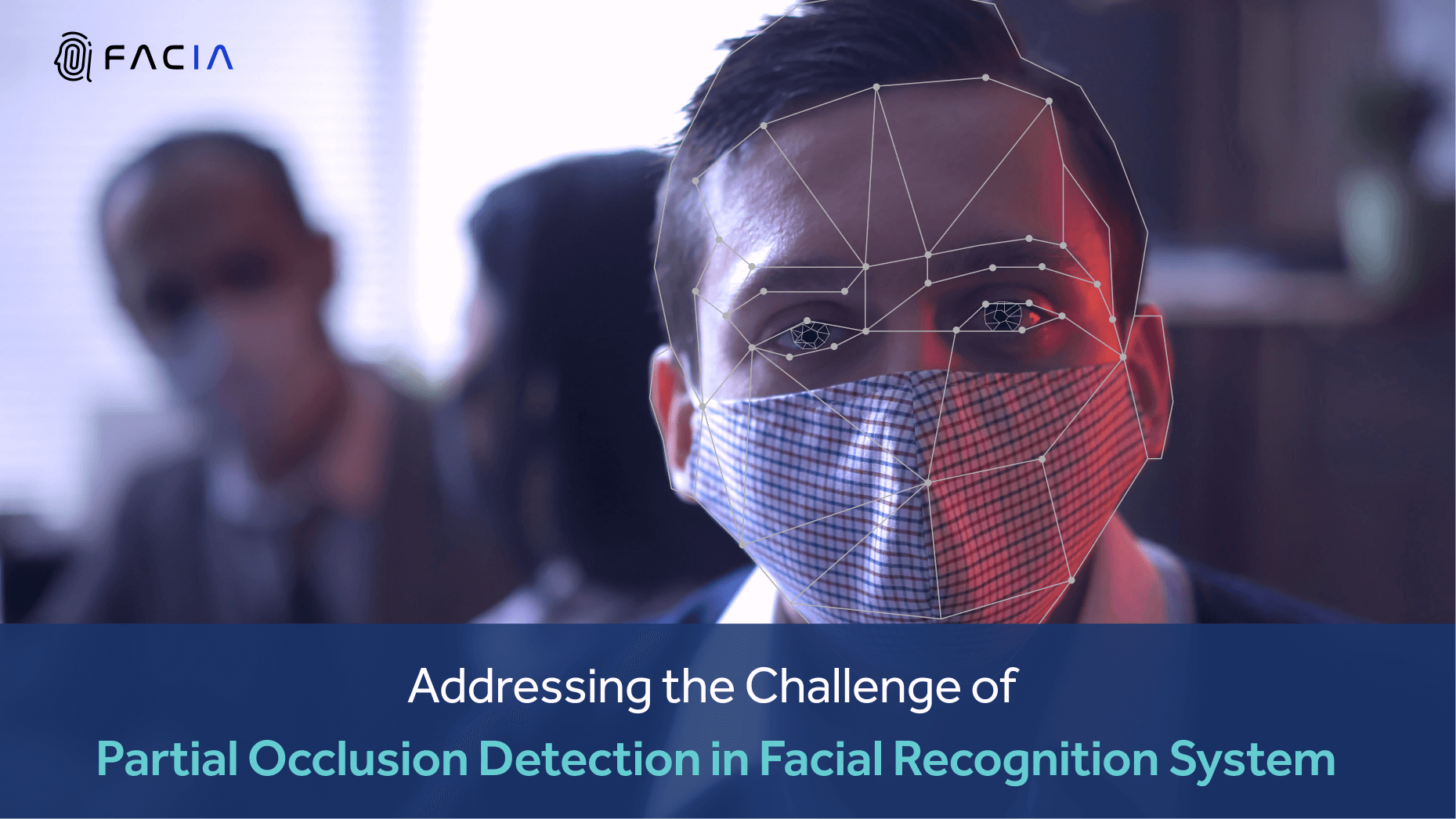
Addressing the Challenge of Partial Occlusion Detection in Facial Recognition System
Author: admin | 23 Jul 2024In This Post
People love to take pictures wearing trendy sunglasses or hats. They also love to add artistic effects and objects in images that may cover some area of their face in pictures. Especially when the COVID-19 pandemic hit, everyone was seen wearing a mask and suddenly people realized that this technique could trick facial recognition systems. This obstruction known as partial facial occlusion in recognizing faces is due to objects and digital artifacts causing failure in facial recognition regarding face matching and identity verification due to incomplete facial datasets.
This blog will address the challenge of partial occlusion in facial recognition and ways to solve it.
Key Takeaways
- Facial occlusion causes increased inaccuracies and error rates in facial recognition under different categories.
- Partial occlusion negatively impacts facial recognition by decreasing its accuracy by almost 9% in some cases.
- Occluded facial recognition is highly important to ensure the detection of genuine users and potential threats.
- Selfie-based facial recognition with partial occlusions is possible with Facia, a
Defining Facial Occlusion
Facial Occlusion means an extraneous object that hinders the view of a face, for example, a beard, a scarf, sunglasses, or a mustache covering lips. Occlusion may occur due to other objects like boxes or a vase appearing in front of the face while taking a picture.
Categories of Facial Occlusion
Different objects create occluded facial images resulting in poor facial recognition. Look at the categories of facial recognition in which different common life objects and other obstructions may occur.
Category | Occlusions |
Facial Accessories |
|
External Occlusions |
|
Limited view |
|
Self-occlusion |
|
Varied lighting conditions |
|
Artificial Occlusion |
|
Impact of Occlusion on Facial Recognition
According to The Ohio State University College of Engineering’s research, facial occlusions covering the eyes caused a drop in facial recognition accuracy from 92% to 37% whereas the lower chin and mouth occlusions caused an inaccuracy rate increase of 8.2%. The author of this research also recommends a face alignment algorithm as a possible remedy for facial feature matching under occlusion but again the problem stays due to the missing datasets.
Why Occluded Facial Recognition is Important?
According to research, facial occlusion is one of the most intractable problems in facial recognition. This is because there is no data on the occluded part before facial recognition is performed on the presented face. Also, collecting large amounts of data on occluded faces and using them as a reference is not feasible to use for deep learning. It is like ‘missing data’ sort of a problem that can’t be recovered by assumptions made by AI or ML models.
Occlusion facial detection is the solution that can identify people with facial occlusion as well. This technology is divided into three approaches:
1. Occlusion Robust Feature Extraction (ORFE)
It searches for the spaces in facial images that are less impacted by occlusions. It employs 2 sub-techniques:
- Patch-based engineered features
- Learning-based features
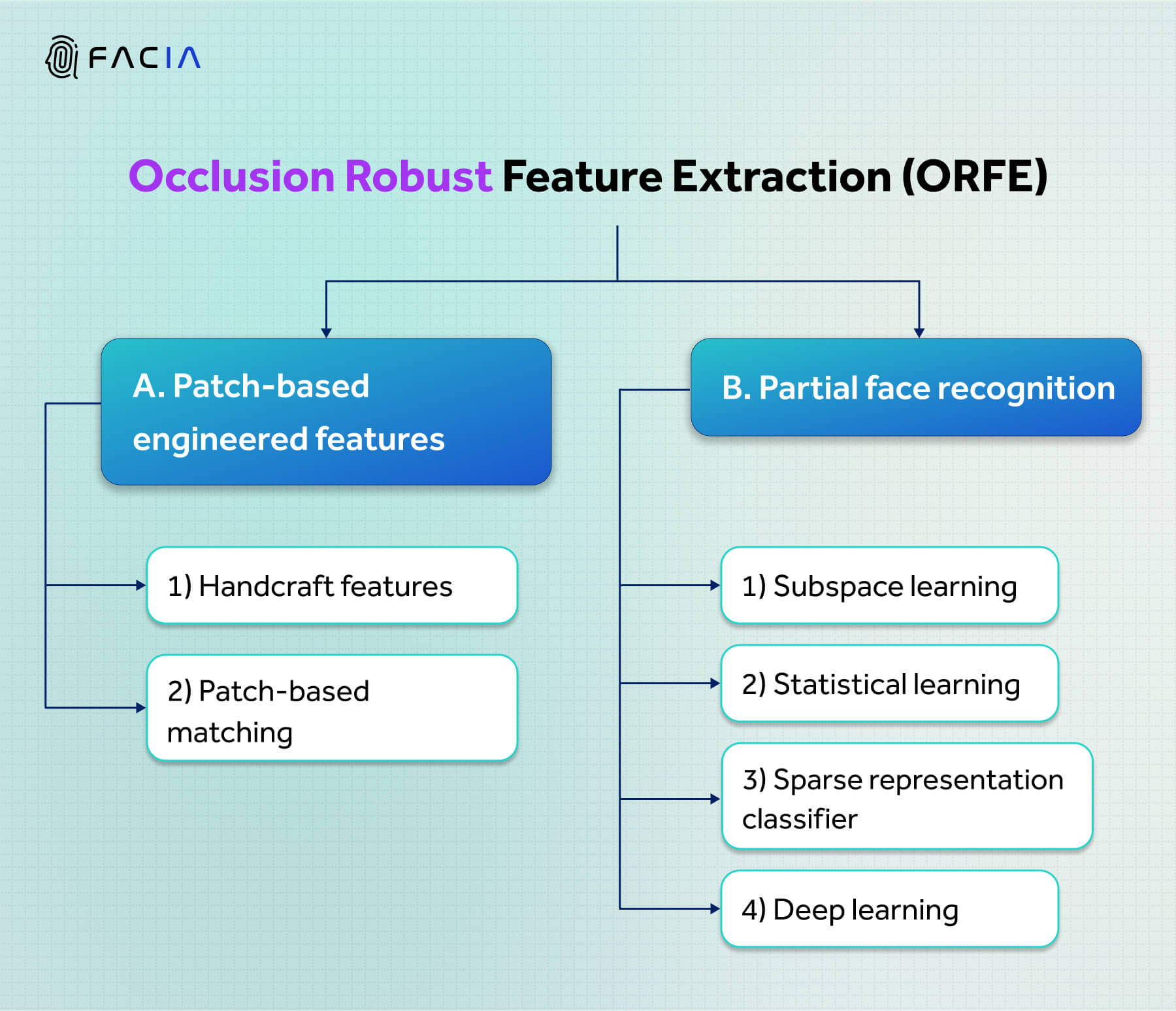
2. Occlusion Aware Facial Recognition (OAFR)
This is by far the most promising category in partial facial recognition occlusion as it discards the occluded part and only considers the visible facial parts for facial recognition. It is aware of the occlusions present in the facial images. It has two goals:
- Feature Extraction
- Face Comparison
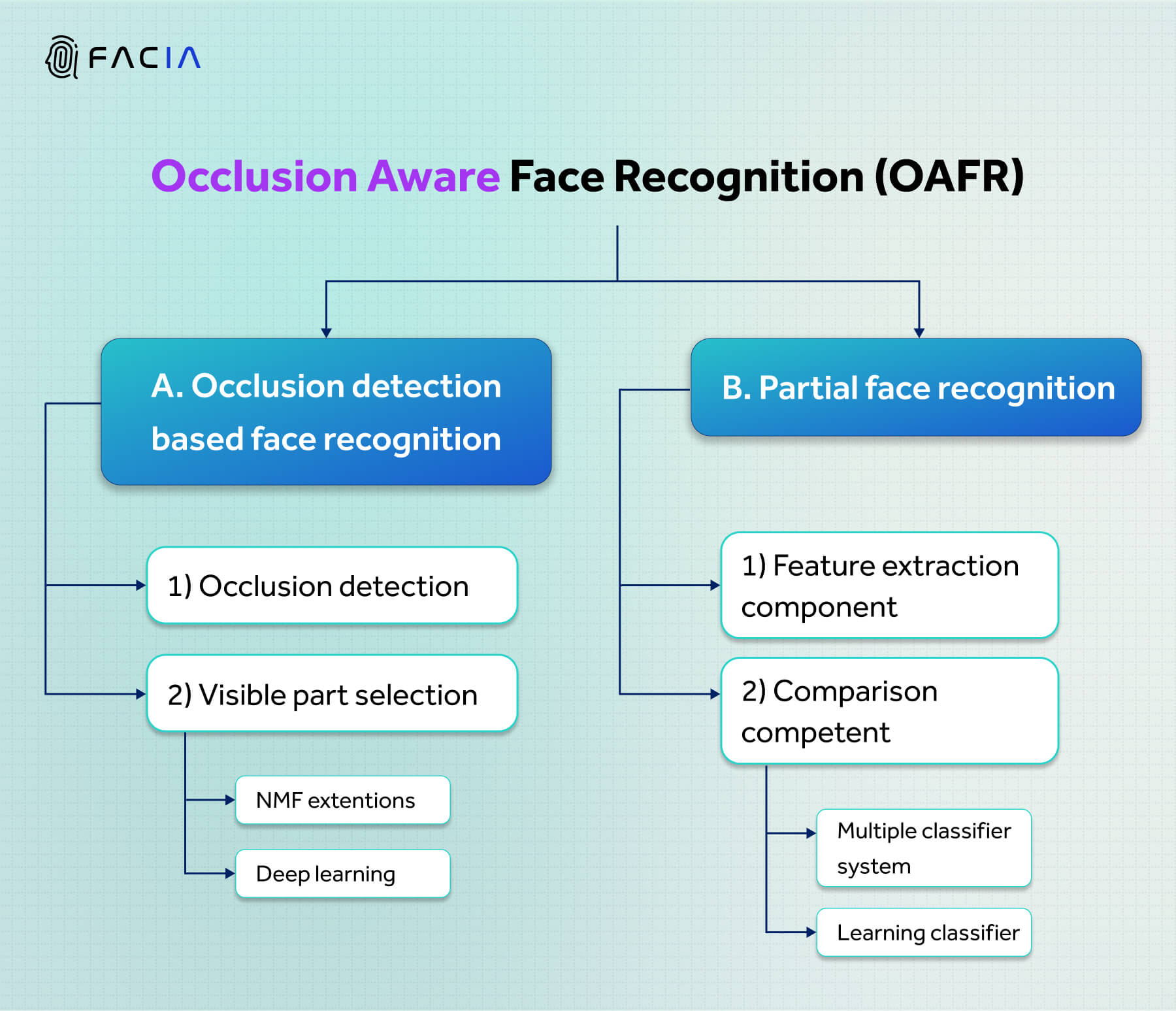
3. Occlusion Recovery-Based Facial Recognition (ORecFR)
This technique focuses on recovering an occlusion-free face from the occluded face to meet the demands of conventional face recognition systems. In other words, it applies an image recovery tactic as the cross-occlusion strategy.
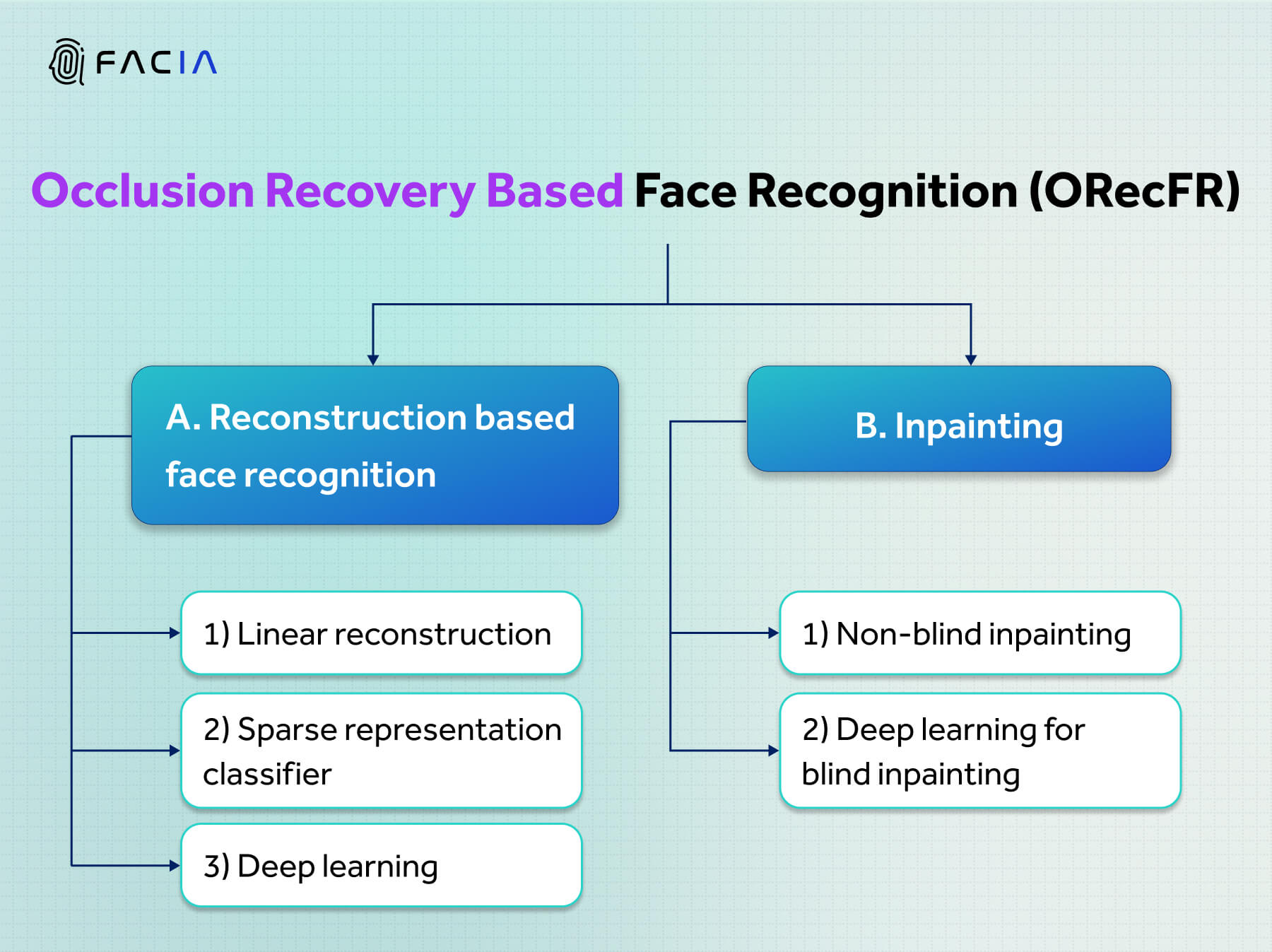
Is Facial Recognition with Occlusions Possible in Real-Time?
Occlusion facial detection is possible with several techniques.
- Robust Algorithms: Deep learning models trained on diverse datasets can carry out facial occlusion analysis with occlusions by focusing on visible features.
- Multi-Frame Analysis: Analyzing multiple frames can be used to reconstruct missing parts (occluded faces) over time.
- 3D Modeling: Using 3D models allows the system to infer occluded areas based on visible features and suggest missing facial parts due to facial occlusions.
- Landmark Detection: Face Recognition Occlusion Systems can identify key landmarks, which are often less affected by occlusion to ensure accuracy.
- Occlusion Detection: Face Detection under Occlusion first detects occlusions and adapts strategies accordingly.
Masked Face Detection and Partial Occlusion Detection
Masked face detection is another technique used in partial occlusion detection that focuses on visible features like the eyes and forehead. Models are trained on datasets that include masked faces, enhancing their ability to recognize partially covered faces. Techniques such as landmark localization and occlusion-resilient models, like convolutional neural networks (CNNs), help detect key features. By analyzing faces at different scales, these systems effectively identify individuals despite the presence of masks. It can further be enhanced by the use of Iris and Retina scanning but then the distance from the camera and clear view of eyes if necessary in this case.
Selfie-based Facial Recognition with Occlusions
Selfie-based facial recognition works on the same pattern. It deals with facial occlusions in the same way with the addition that it can also prompt users to remove a physical occlusion, an artifact applied, or change the brightness and lighting condition. Moreover, the AI-based occlusion correctors may also help in correcting a facial occlusion in facial recognition. It is also important to note that mechanisms like liveness detection in a facial recognition solution may not directly assist the removal of occlusions as their purpose is an entirely different thing.
Facia is an incomparable facial recognition service provider powered by AI focusing on high accuracy rates in the maximum occlusions present. Facia is highly convenient even with the most occluded facial photos and produces facial recognition results with a 0% false acceptance rate at the speed of liveness detection in under 1 second.
Conclusion
Partial Facial Occlusion Detection is an important concern that facial recognition tools must address. It is important to verify identities and detect genuine users with convenience accurately. Occluded facial photos hinder the detection of human faces but the latest facial recognition tools having trained AI and ML models can detect partial faces as well. Users now benefit in terms of speed by not having their sunglasses, beard, or hats removed for a facial recognition check. So far, the accuracy and speed of these tools are still under debate as 100% accuracy is yet to be achieved. However, the solutions are under development, and are hoping to achieve the desired levels of performance.
Frequently Asked Questions
Partial Occlusion in Face recognition refers to an obstruction or a blockage in the face image that can be a wearable gear like sunglasses, a hat, or a beard that partially hinders face image detection.
Facial recognition systems have sophisticated and trained algorithms to detect occlusions and faces behind them. They do it by using probabilistic techniques and predictive analysis of partial occlusions present on a face image.
Partial occlusion hinders facial recognition by hiding essential features like the eyes, nose, and mouth which are crucial for accurate identification of a human face. This leads to uncertainty and increased error rates (FMR and FNMR), as the system struggles with incomplete information. Facial occlusions covering the eyes caused a drop in facial recognition accuracy from 92% to 37% whereas the lower chin and mouth occlusions caused an inaccuracy rate increase of 8.2%. However, with the development of sophisticated algorithms and training on diverse datasets, technology is steadily overcoming these hurdles, improving accuracy and reliability even when parts of the face are obscured.