What is a ROC (Receiver Operating Characteristic) Classifier?
Author: admin | 12 Jul 2024A graphical representation of trade-offs between error rates occurring in biometric systems is important for users as well as decision-makers. Let us discuss the Receiver Operating Characteristic (ROC) Curve which is a pivotal measure of a biometric identity classifier’s performance.
Defining & Explaining ROC Curve
The Receiver Operating characteristic curve is a pictorial representation (through plotting a graph) which is a detailed analysis of a biometric identity verification system in which it demonstrates the trade-off relationship between the True Positive Rate (TPR) and False Positive Rate (FPR). It graphically shows how well a biometric system can differentiate between a genuine user and an imposter. Pertinent to discussing facial biometrics, two main factors are involved in Receiver Operating Characteristic analysis.
ROC is the diagnostic ability of a binary classifier system (facial recognition algorithm) with varying discrimination between an imposter face and a genuine user face.
True Positive Rate (TPR) | The frequency with which a system correctly identifies genuine user faces. |
False Positive Rate (FPR) | The frequency of a biometric system incorrectly identifying imposter faces as genuine users. |
True Negative Rate (TNR) | The frequency with which a biometric system correctly detects and flags an imposter’s face (spoofing attempt). |
False Negative Rate (FNR) | The frequency of a biometric system with which it wrongly detects and flags a genuine user’s face as an imposter. |
Interpretation of an ROC Curve
1. Ideal Receiver Operating Characteristic Curve
An ideal classifier depicts values on an ROC curve that passes through the upper left corner, with a TPR of 1 and an FPR = 0.
2. Random Classifier
In a random classifier, the ROC Curve will be a straight diagonal line from the lower left to the upper right corner of the graph.
3. Enhanced Performance
The ROC Curve closer to the upper left corner is an indication of an enhanced biometric facial recognition system.
4. Area Under the ROC Curve
It indicated the overall performance of the biometric identity solution (classifier). The larger area indicates better discriminative ability.
5. Equal Error Rate (EER)
It is the point of intersection where TPR and FPR are equal.
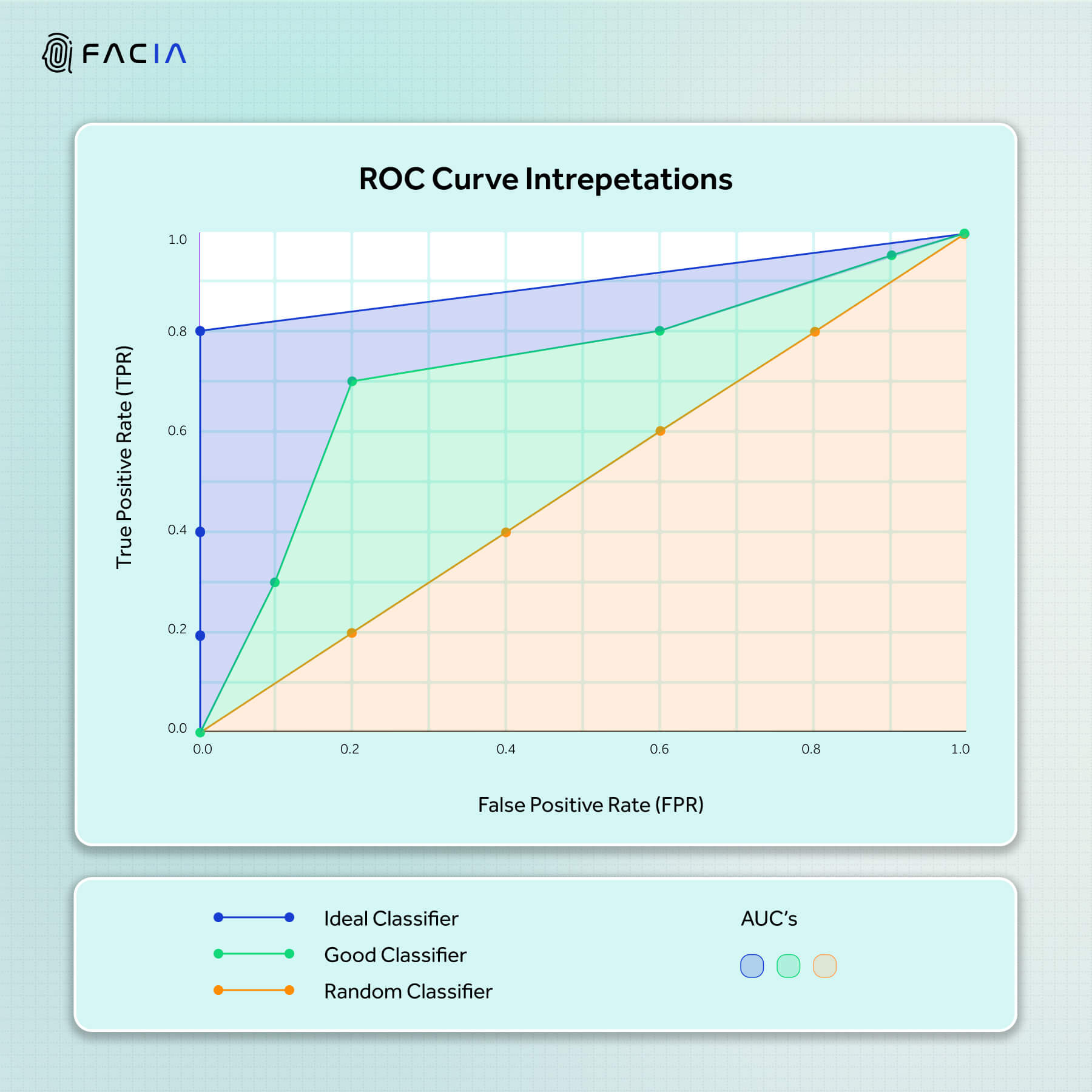
Receiver Operating Characteristic analysis is a valuable tool that helps in comparing biometric identification tools and provides a visual representation of biometric tools across a range of operating points.
Importance of ROC Curve in Facial Recognition
- Performance Evaluation: It provides a comprehensive overview of a facial recognition system’s performance across different threshold settings.
- Threshold Optimization: By analyzing the ROC curve, you can select the optimal threshold that balances the desired trade-off between false positives and false negatives.
- Identity Solution Comparison: ROC curves can be used to compare the performance of different facial recognition algorithms or systems.
How an Ideal ROC Curve can be Achieved?
It is practically unattainable to reach the ideal state in any biometric solution as Ideal ROC is a theoretical benchmark. But face recognition solution providers are striving to reach maximum perfection involving the following factors:
- Improving the Face Image Quality by using well-lit and clear images with minimal occlusions.
- Improving Data Diversity and Data Quantity by encompassing various age groups, genders, ethnicities, and other aspects to improve the datasets.
- Algorithm Optimization by using CNN (Convolutional Neural Networks) and Deep learning to enhance algorithmic accuracy.
- Advanced Feature Extraction of discriminative facial features and identifying the most relevant ones.
- Enhanced Threshold Setting by adjusting threshold according to the specific use case.
Other Considerations
Mitigating the FRT’s biases in datasets and algorithms is critical for achieving a near-ideal ROC. Furthermore, protection against adversarial attacks such as deepfake injection attacks is important to achieve perfection. A user-centric approach alongside improved data quality and implementing AI-based FRT can help in reaching the ideal ROC level.