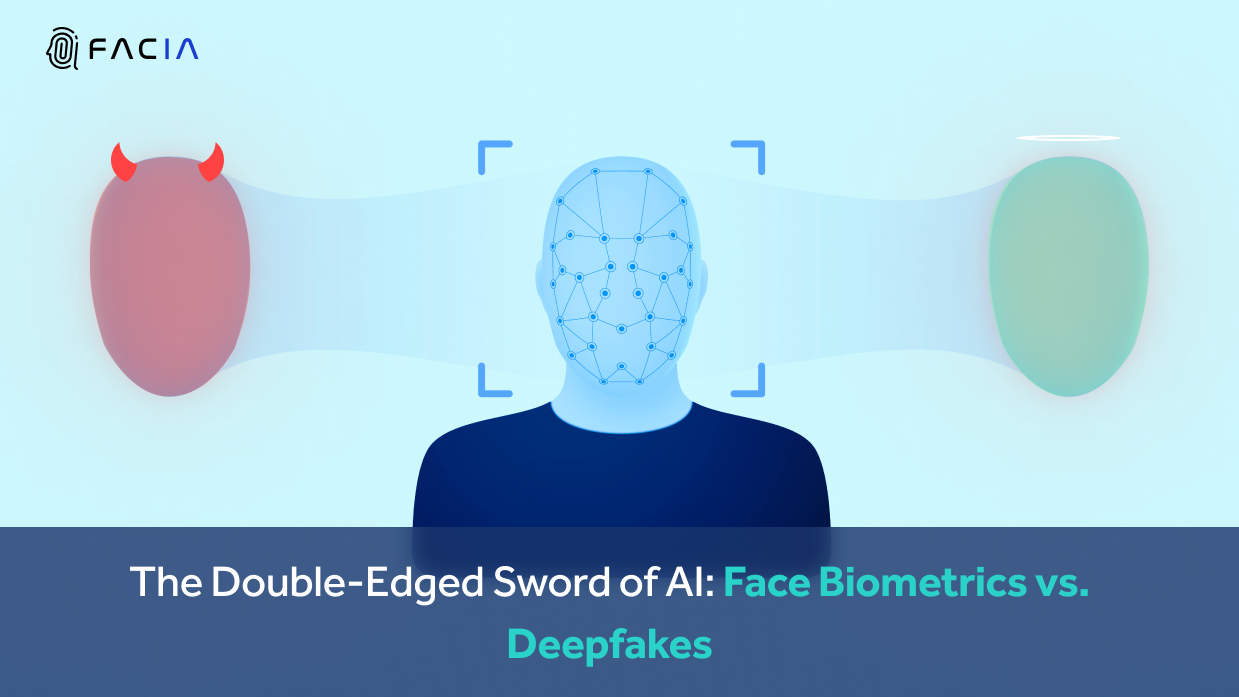
The Double-Edged Sword of AI: Face Biometrics vs. Deepfakes
Author: admin | 04 Jul 2024In This Post
Face biometrics and deepfakes both represent two sides of the same technological coin: both work off of the advanced capabilities enabled by modern artificial intelligence technology. But while one has the goal of enhancing security and individual verification, the other is designed to undermine trust, privacy, and authenticity. This means that the two are and always will be at odds with each other.
But the fact remains that both technologies depend on the same underlying processes to power them. This creates an interesting dynamic that is worth analyzing, especially with the prevalence of these technologies increasing day by day. A report states that the market for artificial intelligence has already passed 184 billion U.S. dollars in 2024, and that number is forecasted to go beyond 800 billion by 2030.
In such a landscape it is important to be aware as the tech evolves how it actually functions in its current implementations. So, let’s discuss the use of artificial intelligence in these two technologies in detail.
The Artificial Intelligence Boom
Artificial intelligence has existed as both a concept and as a piece of tech that has been implemented in one form or another for many many years, yet it has always been more of an abstract concept for regular people. But in the last few years, it feels like out of nowhere, the tech has exploded in popularity, mainly due to innovations in the field creating massive end-user applicability of the technology.
As a result, from conversations with chatbots that feel like talking to a real human being, to generating images based purely off of textual descriptions, to analyzing information in creative ways at lightning-fast speeds; artificial intelligence, it seems, is everywhere.
But among all of the many diverse applications and implementations, two very significant and highly impactful sub-technologies of AI have come to the forefront:
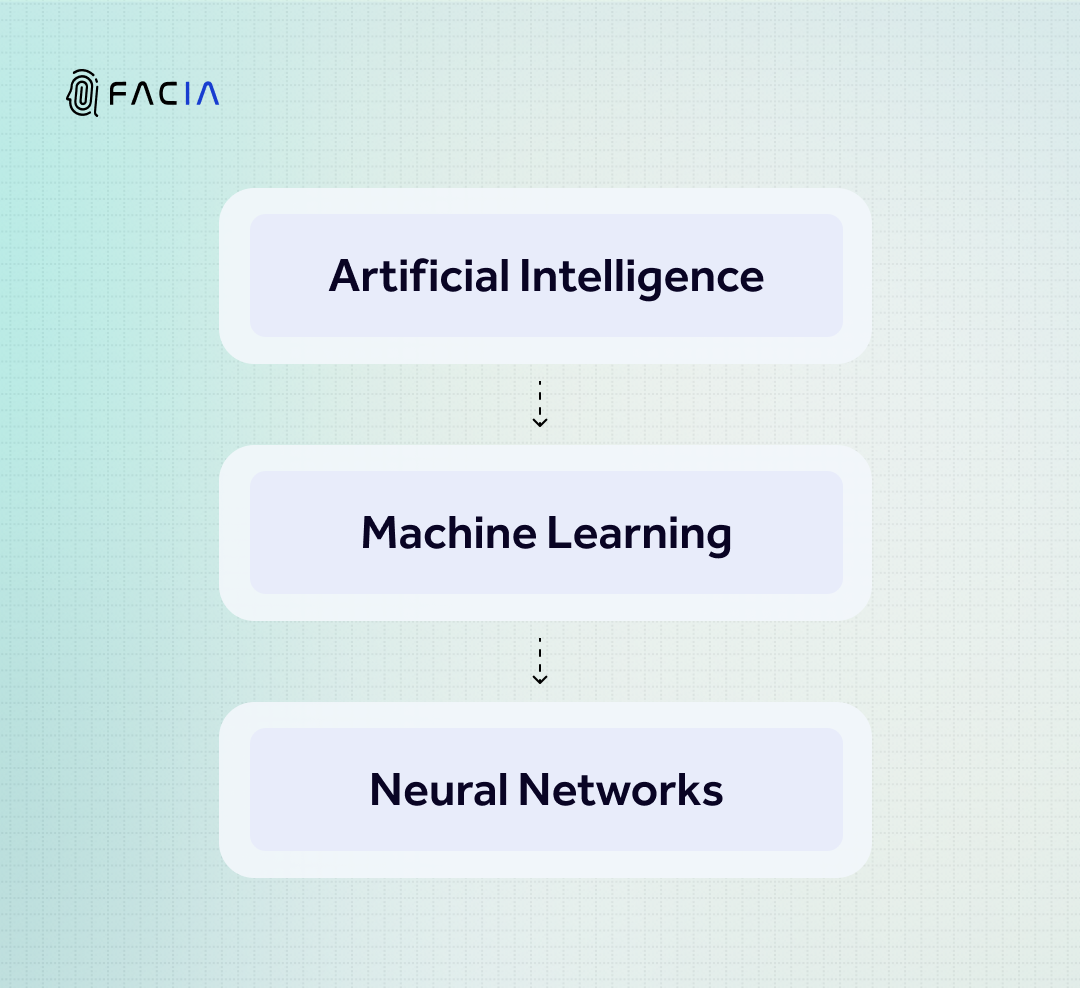
Machine Learning
Machine learning is a kind of artificial intelligence that allows a computer system or program to be able to learn from data, and make decisions, or even predictions, without the need of any direct instructions or input from a human being. The advanced form of this used in facial recognition systems and deepfakes is called ‘deep learning’.
Neural Networks
Neural Networks are a subset of machine learning programs or models that have the capability to make decisions in a similar manner to how a human being would. They emulate the human brain in that they have nodes that work and link together to process information by identifying a phenomenon, weighing the options and possibilities, and then arriving at a conclusion.
How Facial Biometrics Utilizes AI
As the digital world progressed, people realized that conventional mechanisms of securing private systems and spaces, like passwords and PIN codes, were no longer as secure as they once were. These codes could quite easily be hacked, stolen, or sometimes even just guessed.
As a result, modern methods of digital security emerged in the form of biometric authentication and recognition technologies. The most popular of these, due to its ease of use and comparatively wide applicability, is facial biometrics or facial recognition.
Biometric facial recognition utilizes an artificial intelligence-based neural network that is known as a ‘Convolutional Neural Network’ to function.
CNNs (Convolutional Neural Networks)
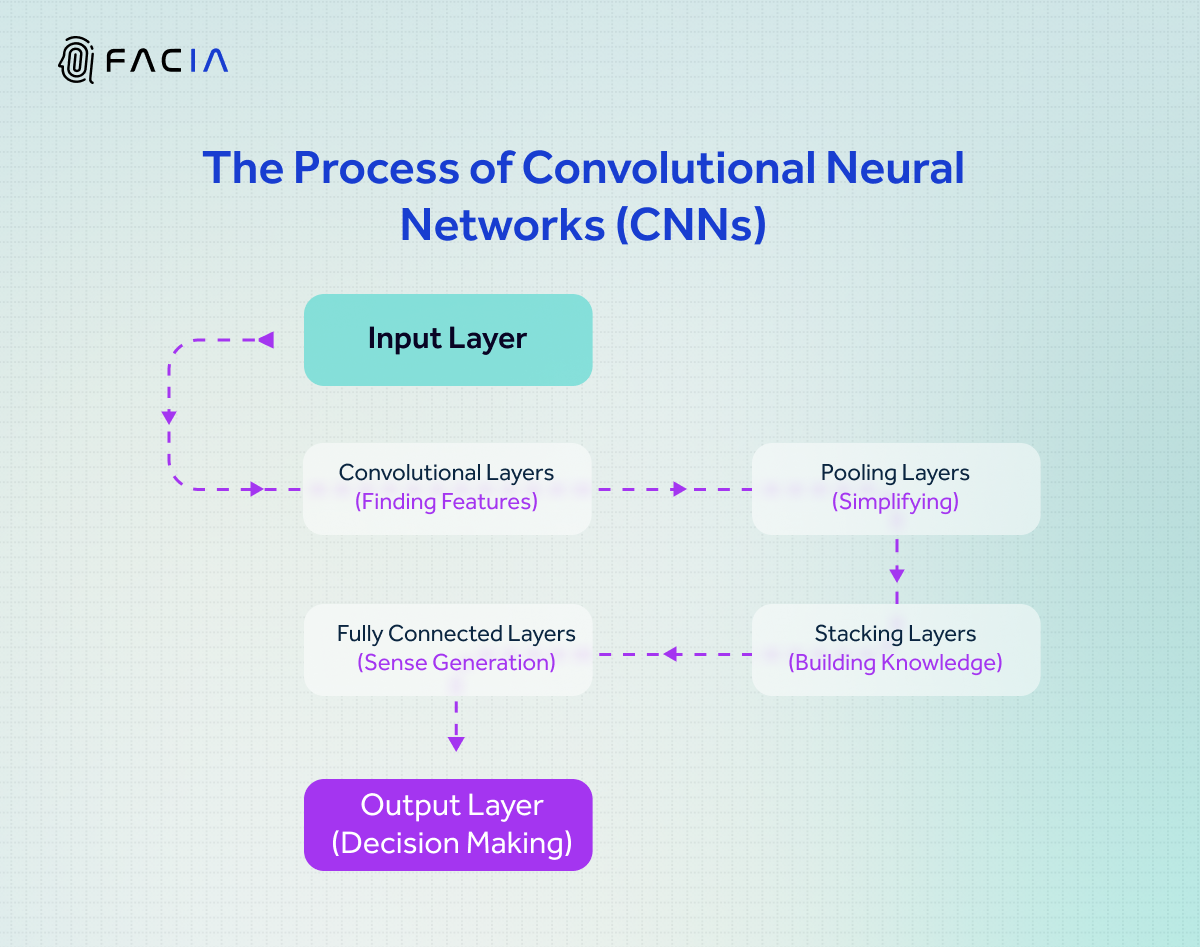
The Convolutional Neural Network is a kind of network that specializes in processing data that has a grid-like structure, such as would be in an image of a face.
The working of this network is structured as layers. The input layer is the starting point, where the system looks at the photo of the face as a combination of pixels with colors and shades. Next comes the titular convolutional layers, where the computer looks for specific patterns in the image using ‘filters’ to find the important features that make up the face, like the eyes, nose, and mouth.
Next are the pooling layers, where the information is simplified so that the focus can be on the most important parts. Afterward come the stacking layers, which is a process of stacking multiples of the previous layers, with the system getting better at understanding the image over the course of the process.
Once sufficient information is gained, the computer needs to make sense of it, which happens in the fully connected layers. The system connects all the details to form a complete understanding of the face. Finally, the system reaches the output layer, where the system makes a decision based on the information it processed.
This process is the core of how a face biometrics process is able to accurately identify a face in different and varied conditions.
How Deepfakes Use AI
While the concept of editing and manipulating images and videos is not new by any means, the innovation of deepfakes was the exceptional level of realism allowed by the use of artificial intelligence technology.
The AI deepfake originated as a simple entertainment tool, where faces of celebrities were added to videos of people performing random, entertaining, or funny acts. But malicious individuals found a use for them to trick biometric recognition technology into allowing them access, by pretending to be an authorized individual.
A special kind of neural network known as a ‘Generative Adversarial Network’ is used in these digital creations.
GANs (Generative Adversarial Networks)
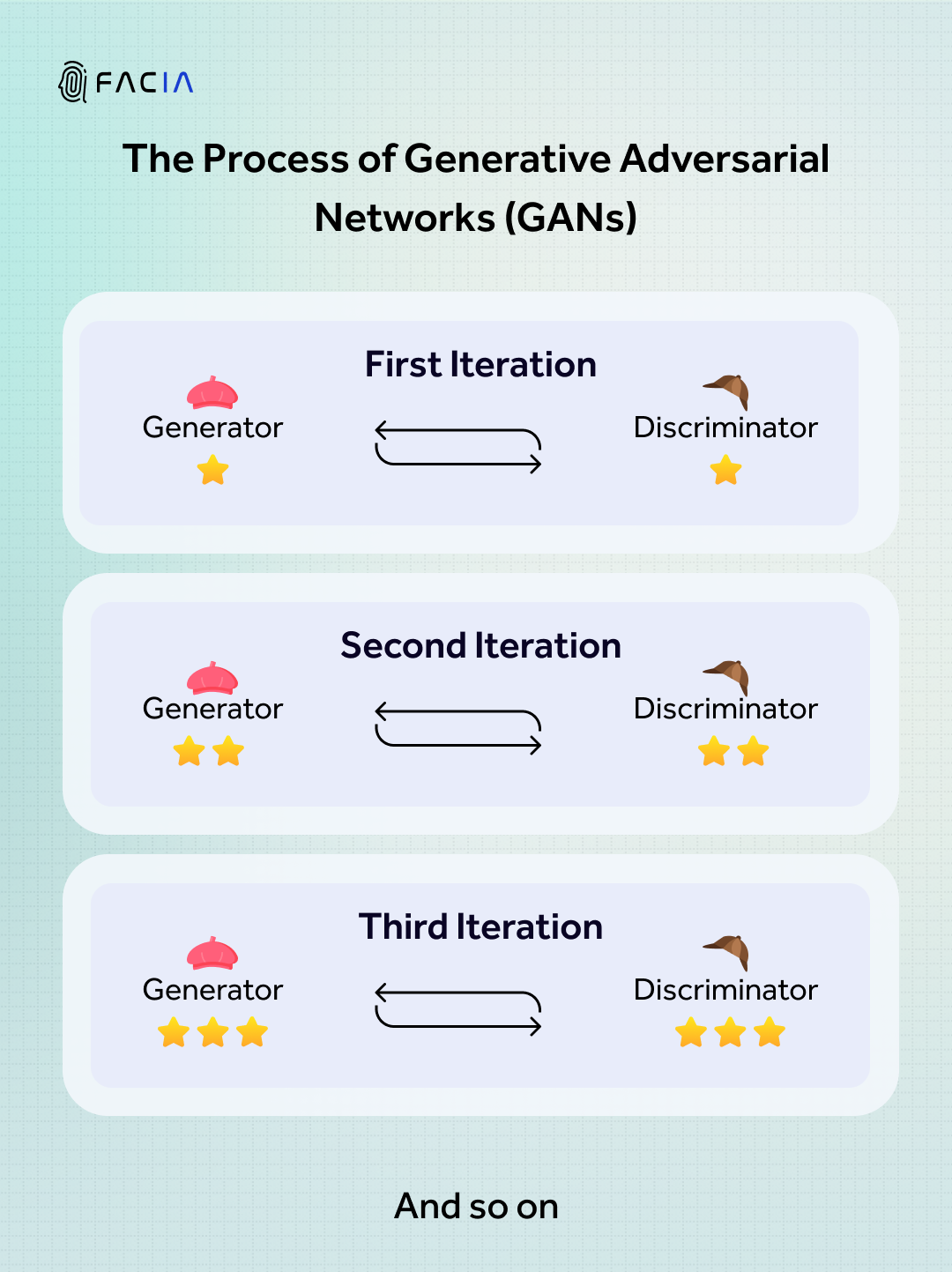
Generative Adversarial Networks are at the core of what allows modern deepfakes to be as realistic as they are.
GANs are actually a combination of two neural networks – a generator and a discriminator. These two neural networks train simultaneously by competing with each other. The goal of the generator is to analyze provided training data, and then use that learned information to generate fake images or videos that imitate the real ones. The discriminator uses that same data to learn how to distinguish between a real and fake image.
So in this process, the generator will create a fake image, and the discriminator will analyze and detect it as a fake. Here, the AI tech allows the systems to analyze what worked, what didn’t, and subsequently how they can improve next time. With each subsequent iteration, the generator creates more and more realistic images, and the discriminator achieves better and better deepfake detection.
Eventually, the generator reaches a point where it can create highly realistic generated imagery that is extremely difficult to determine as fake or real. This is how deepfakes become as realistic looking as they often are.
The Fight Between Facial Recognition & Deepfakes
With the near-simultaneous rise of both facial biometrics and deepfakes, a paradoxical scenario has been created, where the same advancements in AI both provide enhanced security and create new threats.
Interestingly, the situation is quite similar to the GAN networks described above, where each technology is challenging, and subsequently promoting the growth of the other. Scammers and hackers are making deepfakes more and more advanced to trick increasingly sophisticated security systems, and biometric face detection systems are getting more and more advanced to protect from increasingly sophisticated deepfake attacks.
One major innovation thus came to the forefront to fight against this increasing threat of deepfakes:
Liveness Detection
Liveness detection is the groundbreaking innovation that tips the balance unequivocally in favor of biometric security. This piece of tech is able to recognize whether an input provided to a biometric security system is from a live individual, present in the room, or a digital recreation of their credentials. In other words, synthetic rather than biometric data.
With the use of this tech, any biometric security system can be effectively safeguarded against the use of deepfakes, and many other forms of spoofing attacks as well.
Promoting Positive Tech Use with Facia
A major perspective to keep in mind in this conversation about the differing applications of AI technology through face recognition and deepfakes is that one is a positive use and application of modern technology, while the other is a negative, destructive use of tech.
We here at Facia through our solutions promote the positive and productive use of the capabilities of modern technology through our solutions that provide security, trust, and peace of mind. Our 3D liveness detection solutions give you not just security but the mental satisfaction of knowing that your system is secured through some of the most advanced face identification and verification solutions available anywhere in the world.
To get your business, system, or enterprise protected, contact Facia today!
Frequently Asked Questions
Face biometrics are the most popular method of biometric security that have emerged to offer better protection than traditional security mechanisms like passwords. Instead of having to remember complex passwords or PIN combinations, this form of security utilizes your face as an authentication key to provide you access to your account or system.
Face biometrics works using artificial intelligence technology. The system first detects a face inside of an image or camera frame. It then detects and subsequently extracts the features that make up the face including the eyes, nose, mouth and so on, and then uses them to create a digital representation of the face.
It then compares this digital representation against a set of stored faces, and then performs the required action depending on whether or not a match is found.
Face biometrics is one of the most accurate identity recognition solutions currently available, with accuracy rates above 99% percent under ideal conditions. This allows them to be highly applicable for the majority of everyday applications, but the accuracy can be affected by lighting conditions, angles and the image quality of the camera.
Face biometrics are much more secure than conventional methods of digital security and protection like PIN codes and passwords, as there is very little possibility of them being stolen or hacked. When a system of liveness detection is added, facial biometric systems become secure from nearly all kinds of external attacks, like deepfakes and other spoofing attacks.
With how easy to use, convenient and easily applicable to a large variety of situations facial biometrics is, we can expect to see this technology implemented in the majority of industries, situations and spaces that require some form of lock and key mechanism for security. We can also expect to see liveness detection become a fundamental part of the structure of facial recognition-based security systems across the world.